Big Data Myths PersistBig Data Myths Persist
Big data hype has reached a level where some customers are actually avoiding labeling projects as big data, Tibco executives say.
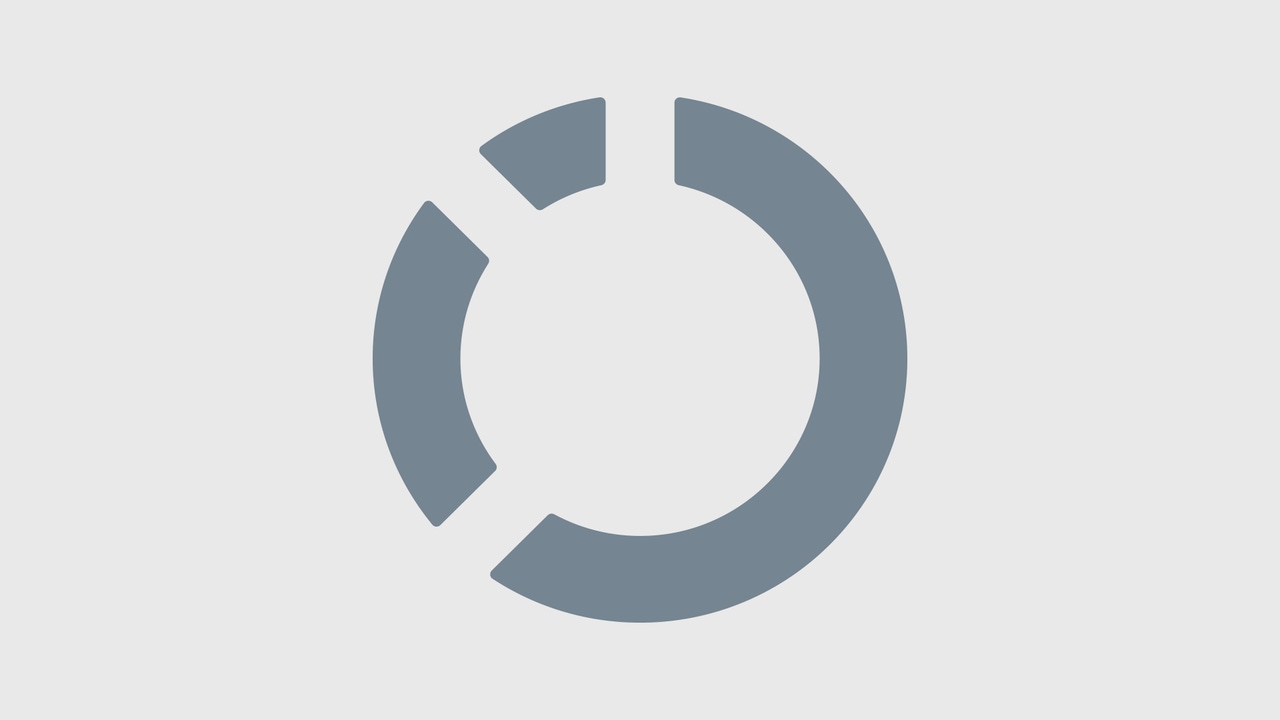
13 Big Data Vendors To Watch In 2013
13 Big Data Vendors To Watch In 2013 (click image for larger view and for slideshow)
The rush to put a "big data" label on every new use of data risks diluting the meaning of the phrase and, worse, may lead some organizations down a disappointing road, say two seasoned IT executives.
"I noticed our customers doing what a lot of people would consider to be 'big data' will often say, "We don't call it big data,'" Chris Taylor of Tibco Software told information in a phone interview. Taylor leads product marketing for business event and in-memory computing products at Tibco.
These customers didn't want their work associated with "needle in a haystack" efforts, and felt such a description was far too limiting, he said.
Also on the call was Taylor's Tibco colleague, company CTO Matt Quinn. A 14-year Tibco veteran, Quinn said it was also important to put big data in a historical context. He said this has been a 20- or 30-year trend of businesses picking out some data, normalizing it and using it to make decisions.
[ Taylor and Quinn will do a deep dive into this topic during Big Data Myths and Real Decisions, at Interop Las Vegas, May 6-10. Use Priority Code DIPR02 by March 2 to save up to $500. ]
"It's a facsimile of a facsimile of a facsimile of what's actually going on out there in the real world," he said. "The challenge people are having at the moment is that business, like life, doesn't fit in nice, clean boxes." Trying to see what's really going on, in all its variety and messiness, "is proving to be a very complex task," he said.
Along with wanting to dispel the idea that data started growing recently, and not over decades, another myth Quinn wants to explode is the well-worn notion of big data's so-called three Vs: volume, velocity and variety.
"I feel a little heretical when I say it, but there's always been variety and a relative amount of volume," he said. "What's happened now is people have started to develop tools to manage and mine that data."
Another myth is that Hadoop is somehow synonymous with big data, Taylor said. "It turns big data into a very batch and offline process," he said, adding this is a mistake because it misses the possibility of managing data "in a real-time scenario."
Another myth: The prevailing idea that these data questions can't be answered without a data scientist on the scene, and that their small number will be a huge constraint on businesses wanting to conduct big data projects.
"I tend to believe this is a misleading discussion," Taylor said. Analytical tools will become more powerful, putting these abilities "into the hands of regular people," he said.
Quinn went further, suggesting a data scientist who lacks business context may actually cause more harm than good.
"This has parallels to the banking crisis," he said. "You had a bunch of quants ... who made some incredibly poor decisions because they didn't have the context."
Rather than becoming enamored of the enabling technologies for big data, Quinn suggested business leaders should first sit back and ask, "What's the No. 1 question that you've wanted to have answered but you were always told it was impossible? Start from there. Don't start with what data you have, start with the important question."
Just as important as finding answers is making them actionable and "operationalizing" them, he added.
Quinn does allow that one category of data, machine-generated data, is exploding. Up until now, companies have typically analyzed just a tiny fraction of any activity.
For example, transactions captured into a database on a retailer's website may represent only 2% of all the server's activity, Quinn said. The rest goes untapped or may be discarded. Now it is possible to analyze this activity, "which may have more value than the transactions" for retailers trying to better model the behavior of visitors, he said.
Not an overhyped myth, according to Quinn, is the impact big data initiatives are having on company organizational structures. "For all the warts and all the good stuff, the lingering success of big data is ultimately going to be measured not in how successful the technology was, but it's going to be how successful the organizational transformation was."
Just like service-oriented architecture before it, Quinn said, the big data revolution is forcing organizational change, including breaking down barriers and silos, and prompting fundamental questions about ownership and governance.
As large healthcare providers test the limits, many smaller groups question the value. Also in the new, all-digital Big Data Analytics issue of information Healthcare: Ask these six questions about natural language processing before you buy. (Free with registration.)
About the Author
You May Also Like