Big Data Success: 3 Companies Share SecretsBig Data Success: 3 Companies Share Secrets
Three C-level execs say start with a clear business goal, consider the data and finish with human-understandable analytics.
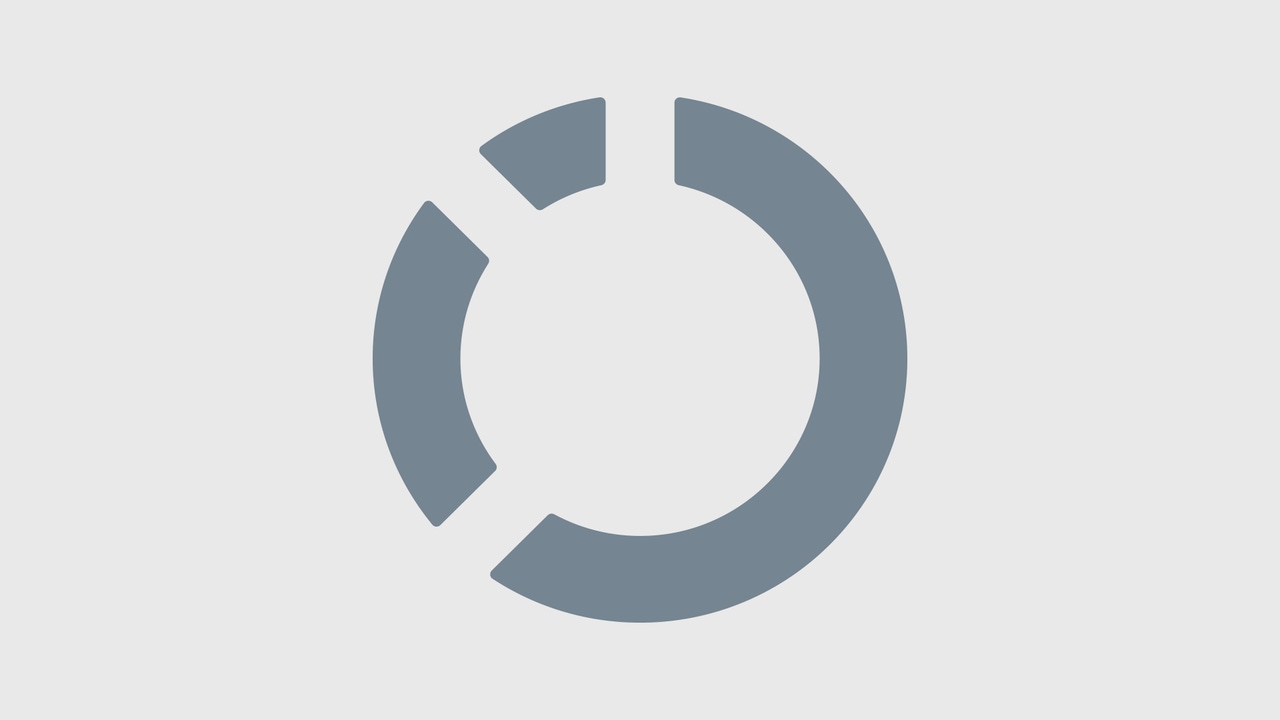
"In our case, all our data resolves to the household level," said Lieberman. "If you don't have some commonality, you end up doing fusion or modeling work that forces you to intuit cause and effect."
TRA knows, for example, that a household was exposed to particular ads running on particular programs and it also knows that that household buys certain products. The company has opted not to use survey data or viewing monitors placed in homes because it can introduce biases, Lieberman said.
At MetLife, global business CIO Hoberman said the company didn't have to be uptight about delivering perfect data through the MetLife Wall project because it was launched internally. A relational database approach with a rigid data model might have delivered perfect results, "but it would have taken years to create the model and products and requirements would change by the time we finished," said Hoberman.
The MetLife Wall was developed and launched in just 90 days, and the data displayed includes confidence scores that alert researchers and customer service reps to the need to validate information.
[ Want more on data-driven insight at airlines? Read Big Data Analysis Drives Revolution In Travel. ]
"Instead of tackling the project with ETL tools and batches, we brought everything into NoSQL so we could show what's critical and score the data," Hoberman said. "Our goal is to expose the Wall for customer self-service eventually, but we're not going to do that until we reach 100% confidence."
Don't Create A Black Box
Any big data deployment is likely to rely on machine learning and advanced algorithms to make sense of the data, but it's important to not let the deployment become too much of a black box.
"As the machine gets smarter, it becomes an alienating force because the output of a machine is very obscure -- it's a score or a mathematical equation and it can appear to be a recommendation without reasoning," said Gupta. "You have to solve the problem of how the decisions are consumed."
Do people understand the underlying logic that drives the decision? Are you making that logic available so humans can make it better? The ultimate goal should be to empower the front-line worker to help the company make superior decisions, Gupta said.
The decision making in digital media and advertising is increasingly automated, executed through machine-to-machine big data analysis, yet it has to remain transparent because "there are still humans there," observed Lieberman of TRA. "There are still CEOs and CMOs who want to know where the media is being bought and the CFO wants to know whether there's a return on the investment."
Indeed, the success of the big data movement will depend on the scaling of big data science so it can be embedded into applications and exposed to ordinary business users who don't have PhDs in statistics. "If we're going to need 500,000 data scientists to make sense of information as some people are saying, big data is doomed to failure," Gupta concluded.
About the Author
You May Also Like