Big Data: The Interdisciplinary VortexBig Data: The Interdisciplinary Vortex
Big data analytics requires companies to operate outside departmental boundaries, and sometimes beyond the spheres of their expertise, to find solutions to certain challenges.
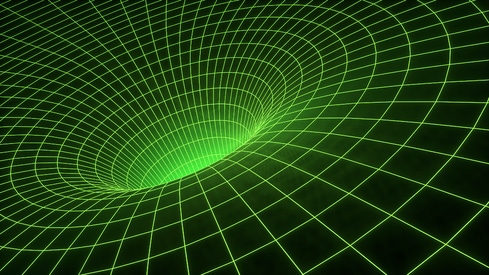
6 Characteristics Of Data-Driven Rock Stars
6 Characteristics Of Data-Driven Rock Stars (Click image for larger view and slideshow.)
Getting the most from data requires information sharing across departmental boundaries. Even though information silos remain common, CIOs and business leaders in many organizations are cooperating to enable cross-functional data sharing to improve business process efficiencies, lower costs, reduce risks, and identify new opportunities.
Interdepartmental data sharing can take a company only so far, however, as evidenced by the number of companies using (or planning to use) external data. To get to the next level, some organizations are embracing interdisciplinary approaches to big data.
Why Interdisciplinary Problem-Solving May Be Overlooked
Breaking down departmental barriers isn't easy. There are the technical challenges of accessing, cleansing, blending, and securing data, as well as very real cultural habits that are difficult to change.
Today's businesses are placing greater emphasis on data scientists, business analysts, and data-savvy staff members. Some of them also employ or retain mathematicians and statisticians, although they may not have considered tapping other forms of expertise that could help enable different and perhaps more accurate forms of data analysis and new innovations.
"Thinking of big data as one new research area is a misunderstanding of the entire impact that big data will have," said Dr. Wolfgang Kliemann, associate VP for research at Iowa State University. "You can't help but be interdisciplinary because big data is affecting all kinds of things including agriculture, engineering, and business."
[Tear down the silos. See How Corporate Culture Impedes Data Innovation.]
Although interdisciplinary collaboration is mature in many scientific and academic circles, applying non-traditional talent to big data analysis is a stretch for most businesses.
But there are exceptions. For example, Ranker, a platform for lists and crowdsourced rankings, employs a chief data scientist who is also a moral psychologist.
"I think psychology is particularly useful because the interesting data today is generated by people's opinions and behaviors," said Ravi Iyer, chief data scientist at Ranker. "When you're trying to look at the error that's associated with any method of data connection, it usually has something to do with a cognitive bias."
Ranker has been working with a UC Irvine professor in the cognitive sciences department who studies the wisdom of crowds.
"We measure things in different ways and understand the psychological biases each method of data creates. Diversity of opinion is the secret to both our algorithms and the philosophy behind the algorithms," said Iyer. "Most of the problems you're trying to solve involve people. You can't just think of it as data, you have to understand the problem area you're trying to solve."
Why Interdisciplinary Problem-Solving Will Become More Common
Despite the availability of new research methods, online communities, and social media streams, products still fail and big-name companies continue to make high-profile mistakes. They have more data available than ever before, but there may be a problem with the data, the analysis, or both. Alternatively, the outcome may fall short of what is possible.
"A large retail chain is interested in figuring out how to optimize supply management, so they collect the data from sales, run it through a big program, and say, 'this is what we need.' This approach leads to improvements for many companies," said Kliemann. "The question is, if you use this specific program and approach, what is your risk of not having the things you need at a given moment? The way we do business analytics these days, that question cannot be answered."
One mistake is failing to understand the error structure of the data. With such information, it's possible to identify missing pieces of data, what the possible courses of action are, and the risk associated with a particular strategy.
"You need new ideas under research, ideas of data models, [to] understand data errors and how they propagate through models," said Kliemann. "If you don't understand the error structure of your data, you make predictions that are totally worthless."
Already, organizations are adapting their approaches to accommodate the growing volume, velocity, and variety of data. In the energy sector, cheap sensors, cheap data storage, and fast networks are enabling new data models that would have been impossible just a few years ago.
"Now we can ask ourselves questions such as if we have variability in wind, solar, and other alternative energies, how does it affect the stability of a power system? [We can also ask] how we can best continue building alternative energies that make the system better instead of jeopardizing it," said Kleinman.
Many universities are developing interdisciplinary programs focused on big data to spur innovation and educate students entering the workforce about how big data can affect their chosen field. As the students enter the workforce, they will influence the direction and culture of the companies for which they work. Meanwhile, progressive companies are teaming up with universities with the goal of applying interdisciplinary approaches to real-world big data challenges.
In addition, the National Science Foundation (NSF) is trying to accelerate innovation through Big Data Regional Innovation Hubs. The initiative encourages federal agencies, private industry, academia, state and local governments, nonprofits, and foundations to develop and participate in big data research and innovation projects across the country. Iowa State University is one of about a dozen universities in the Midwestern region working on a proposal.
In short, interdisciplinary big data problem-solving will likely become more common in industry as organizations struggle to understand the expanding universe of data. Although interdisciplinary problem-solving is alive and well in academia and in many scientific research circles, most businesses are still trying to master interdepartmental collaboration when it comes to big data.
[Did you miss any of the information Conference in Las Vegas last month? Don't worry: We have you covered. Check out what our speakers had to say and see tweets from the show. Let's keep the conversation going.]
About the Author
You May Also Like