Causal AI: AI Confesses Why It Did What It DidCausal AI: AI Confesses Why It Did What It Did
Causal artificial intelligence focuses on understanding cause and effect rather than recognizing data patterns.
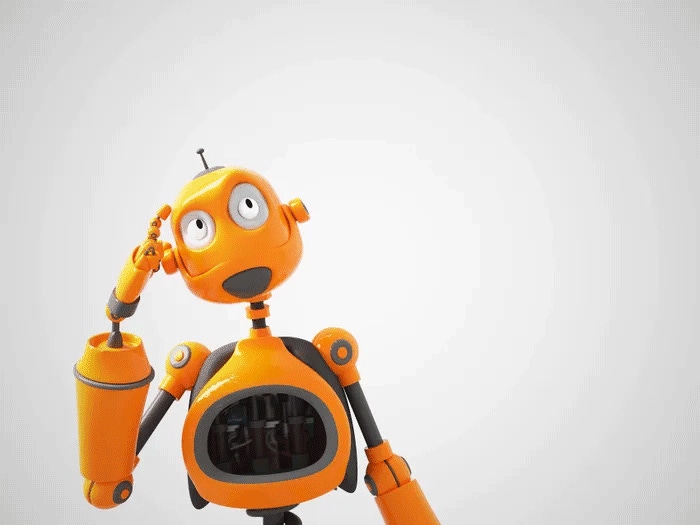
The holy grail in AI development is explainable AI, which is a means to reveal the decision-making processes that the AI model used to arrive at its output. In other words, we humans want to know why the AI did what it did before we staked our careers, lives, or businesses on its outputs.
“Causal AI requires models to explain their prediction. In its simplest form, the explanation is a graph representing a cause-and-effect chain,” says George Williams, GSI Technology’s director of ML, data science and embedded AI. “In its modern form, it’s a human understandable explanation in the form of text,” he says.
Typically, AI models have no auditable trails in its decision-making, no self-reporting mechanisms, and no way to peer behind the cloaking curtains of increasingly complicated algorithms.
“Traditional predictive AI can be likened to a black box where it’s nearly impossible to tell what drove an individual result,” says Phil Johnson, VP data solutions at mPulse.
As a result, humans can trust hardly anything an AI model delivers. The output could be a hallucination -- a lie, fabrication, miscalculation, or a fairytale, depending on how generous you want to be in labeling such errors and what type of AI model is being used.
“GenAI models still have the unfortunate side-effect of hallucinating or making up facts sometimes. This means they can also hallucinate their explanations. Hallucination mitigation is a rapidly evolving area of research, and it can be difficult for organizations to keep up with the latest research/techniques,” says Williams.
On the other hand, that same AI model could reveal a profound truth humans cannot see because their view is obscured by huge volumes of data.
Like the proverbial army of monkeys pounding on keyboards may one day produce a great novel, many crowds of humans may one day trip across an important insight buried in ginormous stores of data. Or we can lean on the speed of AI to find a useful answer now and focus on teaching it to reveal how it came to that conclusion. The latter is far more manageable than the former.
Breaking the AI Black Box
If one gets anything out of the experience of working with AI, it should be the re-discovery of the marvel that is the human brain. The more we fashion AI after our own brains, the more ways we find it a mere shadow of our own astounding capabilities.
And that’s not a diss on AI, which is a truly astounding invention and itself a testament to human capabilities. Nonetheless, the creators truly want to know what the creation is actually up to.
“Most AI/ML is correlational in nature, not causal,” explains David Guarrera, EY Americas generative AI leader. “So, you can’t say much about the direction of the effect. If age and salary correlate, you don’t technically know if being older CAUSES you to have more money or money CAUSES you to age,” he says.
Most of us would intuitively agree that it’s the lack of money that causes one to age, but we can’t reliably depend on our intuition to evaluate the AI’s output. Neither can we rely on AI to explain itself -- mostly because it wasn’t designed to do so.
“In many advanced machine learning models such as deep learning, massive amounts of data are ingested to create a model,” says Judith Hurwitz, chief evangelist, Geminos Software and author of Causal Artificial Intelligence: The Next Step in Effective Business AI. “One of the key issues with this approach to AI is that the models created by the data cannot be easily understood by the business. They are, therefore, not explainable. In addition, it is easy to create a biased result depending on the quality of the data used to create the model,” she says.
This issue is commonly referred to as AI’s “black box.” Breaking into the innards of an AI model to retrieve the details of its decision-making is no small task, technically speaking.
“This involves the use of causal inference theories and graphical models, such as directed acyclic graphs (DAGs), which help in mapping out and understanding the causal relationships between variables,” says Ryan Gross, head of data and applications at Caylent. “By manipulating one variable, causal AI can observe and predict how this change affects other variables, thereby identifying cause-and-effect relationships.”
How Causal AI Works
Traditional AI models are fixed in time and understand nothing. Causal AI is a different animal entirely.
“Causal AI is dynamic, whereas comparable tools are static. Causal AI represents how an event impacts the world later. Such a model can be queried to find out how things might work,” says Brent Field at Infosys Consulting. “On the other hand, traditional machine learning models build a static representation of what correlates with what. They tend not to work well when the world changes, something statisticians call nonergodicity,” he says.
It’s important to grok why this one point of nonergodicity is such a crucial difference to almost everything we do.
“Nonergodicity is everywhere. It’s this one reason why money managers generally underperform the S&P 500 index funds. It’s why election polls are often off by many percentage points. Commercial real estate and global logistics models stopped working about March 15, 2020, because COVID caused this massive supply-side economic shock that is still reverberating through the world economy,” Field explains.
Without knowing the cause of an event or potential outcome, the knowledge we extract from AI is largely backward facing even when it is forward predicting. Outputs based on historical data and events alone are by nature handicapped and sometimes useless. Causal AI seeks to remedy that.
“Causal models allow humans to be much more involved and aware of the decision-making process. Causal models are explainable and debuggable by default -- meaning humans can trust and verify results -- leading to higher trust,” says Joseph Reeve, software engineering manager at Amplitude. “Causal models also allow human expertise through model design to be leveraged when training a model, as opposed to traditional models that need to be trained from scratch, without human guidance,” he says.
Can causal AI be applied even to GenAI models? In a word, yes.
“We could use causal AI to analyze a large amount of data and pair it with GenAI to visualize the analysis using graphics or explanations,” says Mohamed Abdelsadek, EVP data, insights, and analytics at Mastercard. “Or, on the flip side, GenAI could be engaged to identify the common analysis questions at the beginning, such as the pictures of damage caused by a natural event, and causal AI would be brought in to execute the data processing and analysis,” he says.
There are other ways causal AI and GenAI can work together, too.
“Generative AI can be an effective tool to support causal AI. However, keep in mind that GenAI is a tool not a solution,” says Geminos Software’s Hurwitz. “One of the emerging ways that GenAI can be hugely beneficial in causal AI is to use these tools to analyze subject matter information stored in both structured and instructed formats. One of the essential areas needed to create an effective causal AI solution is the need for what is called causal discovery -- determining what data is needed to understand cause and effect,” she says.
Does this mean that causal AI is a panacea for all of AI or that it is an infallible technology?
“Causal AI is a nascent field. Because the technology is not completely developed yet, the error rates tend to be higher than expected, especially in domains that don’t have sufficient training for the AI system,” says Flavio Villanustre, global chief information security officer of LexisNexis Risk Solutions. “However, you should expect this to improve significantly with time.”
So where does causal AI stand in the scheme of things?
“In 2022 Gartner Hype Cycle, causal AI was deemed as more mature and ahead of generative AI,” says Ed Watal, founder and principal at Intellibus. “However, unlike generative AI, causal AI has not yet found a mainstream use case and adoption that tools like ChatGPT have provided over generative AI models like GPT,” he says.
About the Author
You May Also Like