Cognitive Security Tames the Big Data MonsterCognitive Security Tames the Big Data Monster
Sometimes data can get so big, It's monstrous and ceases to be useful. Cognitive security helps measure what matters, allowing analysts to focus resources where they count.
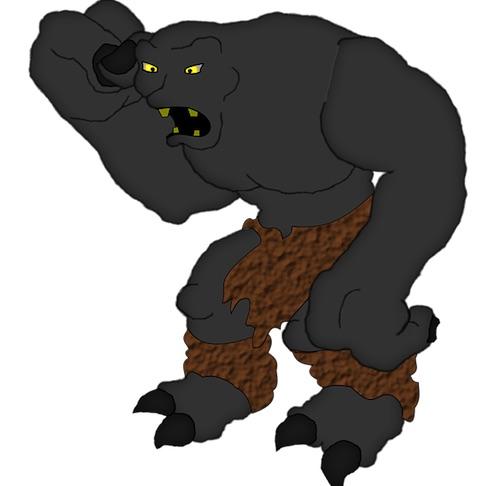
The volume of event data that could be harnessed for security analysis bloats very fast even in small networks causing the risk of resource wastage on the wrong data while possibly missing good information, hence the reason why cognitive security is important.
In data-driven security, the goal should be to measure what matters, as not all data is useful. However, without a real reason to discriminate one set of data over another, the prudent path is to analyze everything. The result of that is strained resources including time, computing, and storage resources.
Cognitive analytics is an interesting subject in this context as it begins to offer a solution to the unwieldiness of big data. There are numerous tools now for the processing of data of various forms, but the problem is how to reduce the search for useful insight to center around what is known to be the most valuable information over time. In that sense, the cognitive security paradigm takes a machine learning approach to data processing to determine which is the data that really matters. In a white paper, IBM describes cognitive security as the implementation of self-learning systems that use data mining to mimic the functioning of the brain.
Cognitive insights, as one example, refers to the algorithm behind its version of cognitive analytics solution as automated signature construction, which as they discuss enables a security system tell when something irregular is happening that could indicate a threat even though the specific event does not match any existing threat signature.
The essence of cognitive analytics is the following: a human analyst can design a logical pattern of correlating and analyzing data and then give it to a machine that can apply this reasoning at a massive scale and also retain memory of the important outcomes for future application. For example, SparkCognition mentions that its artificial intelligence infrastructure can read through billions of pages of manufacturers' instructions and maintenance manuals. If an AI system can have access to this type of data in its complete form and for all components of a large system, then it can form correlations among possible causes of defects and failure in one component with possible sets of behavior in another component.
As a result, whenever actual behavior data starts flowing in, this AI analyst system can identify data that identifies potentially important relationships immediately to flag possible threats and potential failure. Additionally, with the ability to take a component and thoroughly research it in relation to potential threats and failures, cognitive analytics AI can also model potential failures not yet experienced and enabling the system to recognize future events if they begin to follow a potentially risky trajectory.
Cognitive analytics relies on data available within the network and data publicly available from the internet and other sources to continuously model threat patterns. This data includes attacks, exploits, threat signatures, solutions, threat evolution patterns, and other details of anomalous behavior in networks as well as data on different system components, their manufacturing, variation of models, failure patterns, and unsolved problems. All this data can then be applied with human expert-like reasoning while operating at large scale to cut through tons of data that would otherwise be hard to make optimal use of because of sheer volume.
At this level of analytics, data that is not meaningful can be identified as such immediately, and even though it may be processed or preserved in some way it doesn't present a risk of diverting useful resources towards analysis of useless data at crucial points in time. Even useful data can be analyzed based on known previous patterns in the sense that where the outcome of processing such data is always the same then previous information can be utilized while it remains reasonable. Over time cognitive analytics usage might make a big difference in helping organizations determine detect a threat in time, one moment too late, or never.
Read more about:
2017About the Author
You May Also Like