Defining an AI Governance PolicyDefining an AI Governance Policy
Regulators still lack a firm grip on what AI governance should do, but that’s no reason for IT departments to wait to make use of AI.
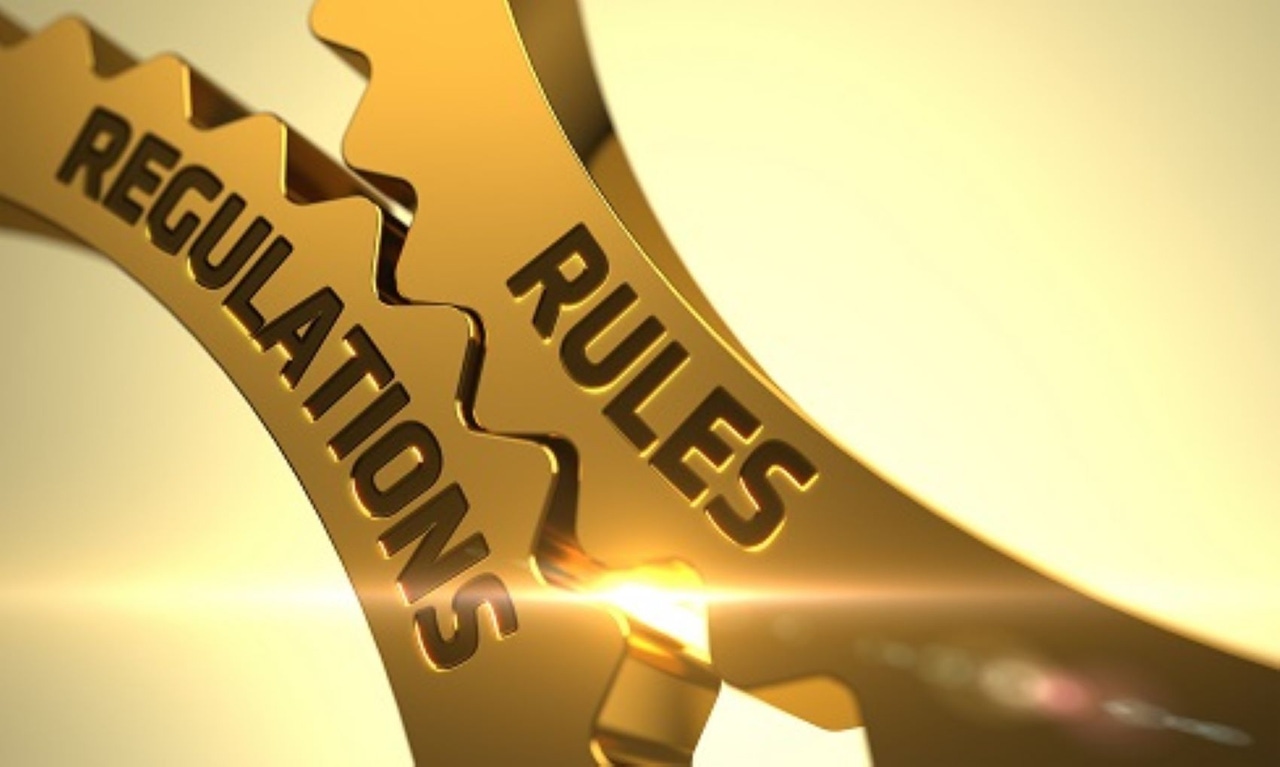
Every company knows it needs an AI governance policy, but there’s scant guidance for creating one. What are the crucial issues, and how do you begin?
There are AI governance outlines and templates everywhere, but no one, not even regulators, government officials or legal experts, knows the situations where AI will require guidance and governance. This lack of understanding is attributable to the newness of AI.
Since there is so much AI governance uncertainty, many companies are passing on defining governance, even though they are investigating and implementing AI in their businesses. I’m going to argue that companies don’t have to wait to define AI governance. They can begin with what they already know from privacy, anti-bias, copyright and other regulations, and start by incorporating these known elements into an AI governance policy.
Here’s a summary of what we already know.
Privacy
Privacy laws can vary from state to state and from country to country. What we do know is that individuals have the right to personal privacy, and the right “to be left alone” under US law. Individual data is highly confidential, particularly in the healthcare and financial fields. Individuals must sign privacy statements agreeing to the sharing of their information with certain third parties, if the information is to be shared. Privacy policies also explain what information companies will protect.
Applying these basics to AI, this means that patient data, as one example, is likely to be anonymized if it is being grouped into a demographic of individuals with a propensity for a particular disease or condition. So, for a medical diagnostics AI system that is being used to arrive at a diagnosis for a particular patient, the AI algorithm can investigate summary data on patients that it has on file, but it can’t delve into the particulars of any one of the patients whose data has been aggregated or it will risk violating the patient’s privacy rights.
Anti-Bias
Discrimination and bias are integral parts of employee law that should be formalized in AI governance.
Organizations have already experienced AI miscues from bias by not populating their systems with sufficiently unbiased data, and by developing faulty algorithms and queries.
The result has been seriously biased systems that returned inaccurate and embarrassing results. This is why diversity and inclusion should be integral to AI work teams, in addition to reviewing the data to ensure that it is as free from bias as possible.
Diversity applies to the makeup of AI employees, but it also applies to company departments.
For instance, finance might want to know how to improve product profit margins, but sales might want to know about how to improve customer loyalty, and engineering and manufacturing might want to know about how to improve product performance so there are fewer returns. Collectively, all of these perspectives should be included in an AI analysis of customer satisfaction, or you risk getting biased and inaccurate results.
“One of the biggest risks in AI is the replication of existing societal biases. AI systems are only as good as the data they are trained on, and if that data reflects biased or incomplete worldviews, then AI’s outputs will follow suit,” noted Nichol Bradford, executive in residence for AI+HI at the Society of Human Resource Management.
Intellectual Property
Generative AI paves the way for others’ visual and word-based creations to be collected and re-purposed for use, often without the company’s or the originator’s knowledge. For example, your company could enter into an agreement with a third-party vendor whose data you want to buy for your AI data repository. You cannot be sure of how the third party obtained their data, or if their data is potentially violating copyright or intellectual property law.
The Harvard Business Review discussed this issue in 2023. It stated, “While it may seem like these new AI tools can conjure new material from the ether, that’s not quite the case … This process comes with legal risks, including intellectual property infringement. In many cases, it also poses legal questions that are still being resolved. For example, does copyright, patent trademark infringement apply to AI creations? Is it clear who owns the content that generative AI platforms create for you, or your customers? Before businesses can embrace the benefits of generative AI, they need to understand the risks -- and how to protect themselves.”
Unfortunately, it’s hard to understand what the risks are because intellectual property (IP) and copyright infringements in AI are just beginning to be challenged in the courts, and case law precedents have yet to be established.
Until legal clarifications can be made, it’s advisable for companies to initially draft governance guidelines for IP and copyrights that stipulate that any vendor from whom data is purchased for use in AI must be vetted and warrant that the data offered is free from copyright or IP risks. Internally, IT should also vet its own AI data for any potential IP or copyright infringement issues. If there is data that could pose an infringement problem, one approach is to license it.
Establishing AI Governance in the Organization
It will fall to the IT team to start the AI governance process. This process must begin with dialogues with the C-suite and the board. These key stakeholders must support the idea of AI governance in action as well as in words, because AI governance will affect employee behaviors as well as data and algorithm stewardship.
The most likely departmental AI “landing spots” must be identified because those departments will be most directly responsible for subject matter expert input and AI model training, and they will need training in governance.
To do this, an interdepartmental AI governance committee that agrees to governance policies and practices should be formed. It should have committed executive leadership backing it.
AI governance policy development will be fluid because AI regulation is fluid, but organizations can begin with what they already know about privacy, intellectual property, copyrights, security and bias. These initial AI governance policies should be accompanied by training for the internal employees who will be working with AI.
What’s presently important for CIOs is making AI governance an integral part of AI system deployment. There is every reason to do this now with what we already know about sound data handling practices.
About the Author
You May Also Like