Emotion Detection in Tech: It’s ComplicatedEmotion Detection in Tech: It’s Complicated
The advancement of human-machine partnerships requires emotion detection and appropriate responses in context, but it's a tough problem.
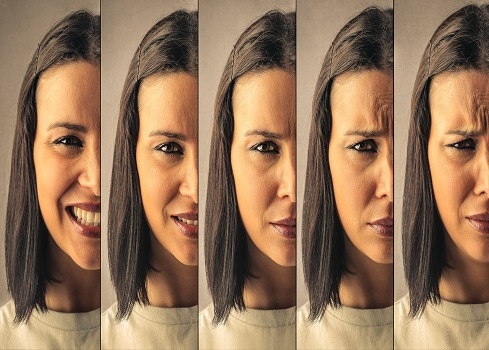
Of all the potential types of analytics, emotion analytics is one of the toughest to perfect because human emotions are complex. For example, there are genuine reactions and fabricated ones as well as cultural and individual differences that shape our perceptions and behaviors. There are also other things to consider such as context. While emotion analytics is clearly important to the future of analytics, AI, robotics, intelligent automation and applications, the early-stage excitement can lead to unrealistic expectations.
"Detecting and classifying emotionality is still a challenging problem," said Manish Kothari, president of research institute SRI International, which is currently developing advanced Emotional Artificial Intelligence automotive technology that will enable the next generation of vehicles to detect drivers' emotions and respond accordingly. "It's easy to detect extreme arousal, extreme happiness or extreme unhappiness, but to detect the subtler elements is still challenging."
Context improves accuracy, such as having a diagnosis or knowledge set indicating that a person is depressed, he said.
Who's adopting emotion analytics
Dan Simion, Capgemini North America’s VP of AI and Analytics said his company is seeing more adoption among consumer-facing clients in the media, entertainment, retail and travel and hospitality industries.
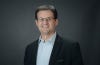
Dan Simion, Capgemini
For example, Capgemini worked with a media provider to detect the emotion of a live in-studio audience. Using a live camera feed to monitor the audience, they could understand which segments of the shows received more positive responses than others and tailor the pace of the shows and segments. Even the topics could be adjusted to increase audience engagement.
Chatbots seem like an obvious use case, but Simion said organizations are more interested in implementing them to reduce costs than emotion analytics. Other areas of slow traction include B2B companies and focus groups.
Simion said cruise ships are using security camera feeds to monitor guests' emotions as the participate in different activities.
How to analyze emotion
Understanding emotions involves analyzing verbal and non-verbal clues.
"The optimal pathway would be to use all three specific modalities," said SRI's Kothari. "One would be computer vision because facial gestures, body gestures and body language communicate a lot. The second is voice intonation and the third is the words themselves."
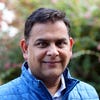
Manish Kothari, SRI International
Emotion detection would be a lot easier if humans expressed themselves in homogenous ways. However, cultural backgrounds and unique life experiences influence personal expression.
Michelle Niedziela, VP of research and innovation at market research firm HCD Research, said advertisers and their agencies can get overly excited about the "happy" responses an ad drives when the response may have been a natural reflex.
"If I smile at you, you innately smile back. So, one thing is are they really feeling happy or just projecting happy?" said Niedziela. "But also, how big does a smile have to be in order to be interpreted as happy?"
Even cheap camera sensors are improving, but some of them may not be able to detect subtle nuances in facial geometry or provide the same degree of reliability among individuals who represent different races. Also, things that change an individual's appearance like hats, bangs or facial hair can negatively impact the accuracy of emotion sensing.
"In my mind, the two biggest challenges are hardware quality and the models," said Capgemini's Simion. "You need to be very careful when you're talking about emotionality is the dataset you're going to use because if you're just going to call normal APIs from the cloud providers, that's not going to help much."
Like SRI's Kothari, HCD's Niedziela advocates a holistic approach to emotion analytics.
"If you're studying shampoo, you don't just ask whether [a person] likes it or not. You ask a whole bunch of hedonic questions such as how sticky was it? How much did it foam? What did it smell like? And then you also have emotion and purchasing behavior," said Niedziela. "If you use a Bayesian approach, then you can take all that data, lay it out and see if I were to change the bubble size in the foam, how that's going to drive liking."
Niedziela also captures state changes to determine how something such as a shampoo's scent or a TV ad has affected someone's emotional state.
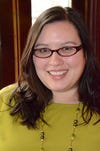
Michelle Niedziela, HCD Research
"If you just measure people without any sort of context changes for baseline or differences between experiences, then it's really hard to say what you're measuring," said Niedziela. "Without that, I might just be measuring that you woke up on the wrong side of the bed that day and that's not as informative."
Emotion analytics capabilities are available now that address the visual, voice and text elements. For example, facial coding solutions are available from iMotions, Visage Technologies and Noldus, while Affectiva combines computer vision, speech analytics and deep learning. Twinworld and ParallelDots both offer a text analysis API. Then on the consumer side, the Amazon HALO fitness wristband analyzes vocal intonation so users can monitor their emotional states throughout the day.
Beware of oversimplifying the problem
The oversimplification of an emotional state could lead to faulty conclusions. For one thing, emotions are not mutually exclusive, which is why people sometimes say they "have mixed feelings" about something. Similarly, a bittersweet experience is both happy and sad. In addition, an individual may react differently to the same stimulus in different contexts.
Oversimplifying analytics is also problematic.
"You should never just rely on neuroscience or some fancy new tool because you need to integrate in other aspects. You still have to ask people to [describe] their experiences," said Niedziela. "There are also a lot of cognitive things going on that are driving behaviors so it's important to understand appreciate and respect that humans are complex, brains are complex."
Other considerations
It also turns out that what works well between humans may not work as well between humans and machines. For example, one reason psychologists repeat what a patient says is to make the patient feel "heard." Law enforcement officers use the same technique to deescalate crisis situations.
Should a CRM chatbot do the same? Yes, but not to the same degree because the context is different. After all, customers contact support to expedite the resolution of a problem. Contrast that with an emotional support robot, which by definition must be able to recognize and respond to human emotions.
Another consideration is the level of accuracy a use case requires.
"If you're [trying to determine] whether a person is suicidal or not and you're going to call 911 automatically, that requires a high level of accuracy," said SRI's Kothari. "If you're trying to determine whether someone is drowsy in a car and you want to give them a burst of cold air if they are, then you're willing to accept a few false positives to avoid the circumstance. Different situations and different actions require different levels of accuracy."
Emotion analytics continues to mature, and the use cases are practically infinite. Eventually, the capabilities will become mainstream as evidenced by a growing number of API calls, emotionally sensitive applications and services, industry partnerships, mergers, acquisitions and other market growth indicators. Verified Market Research estimates that the global emotion analytics market will triple from $1.82 billion in 2018 to $5.46 billion by 2026.
About the Author
You May Also Like