Enterprise AI Goes Mainstream, but Maturity Must WaitEnterprise AI Goes Mainstream, but Maturity Must Wait
An O'Reilly survey illustrates how enterprise groups are moving more applications into production, but also how companies face cultural and talent focused barriers.
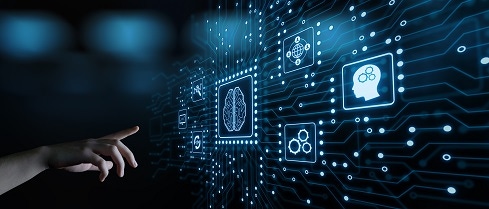
Artificial intelligence’s emergence into the mainstream of enterprise computing raises significant issues -- strategic, cultural, and operational -- for businesses everywhere.
What’s clear is that enterprises have crossed a tipping point in their adoption of AI. A recent O’Reilly survey shows that AI is well on the road to ubiquity in businesses throughout the world. The key finding from the study was that there are now more AI-using enterprises -- in other words, those that have AI in production, revenue-generating apps -- than organizations that are simply evaluating AI.
Taken together, organizations that have AI in production or in evaluation constitute 85% of companies surveyed. This represents a significant uptick in AI adoption from the prior year’s O’Reilly survey, which found that just 27% of organizations were in the in-production adoption phase while twice as many -- 54% -- were still evaluating AI.
From a tools and platforms perspective, there are few surprises in the findings:
Most companies that have deployed or are simply evaluating AI are using open source tools, libraries, tutorials, and a lingua franca, Python.
Most AI developers use TensorFlow, which was cited by almost 55% of respondents in both this year’s survey and the previous year’s, with PyTorch expanding its usage to more than 36% of respondents.
More AI projects are being implemented as containerized microservices or leveraging serverless interfaces.
But this year’s O’Reilly survey findings also hint at the potential for cultural backlash in the organizations that adopt AI. As a percentage of respondents in each category, approximately twice as many respondents in “evaluating” companies cited “lack of institutional support” as a chief roadblock to AI implementation, compared to respondents in “mature” (i.e, have adopted AI) companies. This suggests the possibility of cultural resistance to AI even in organizations that have put it into production.
We may infer that some of this supposed lack of institutional support may stem from jitters at AI’s potential to automate people out of jobs. Daniel Newman alluded to that pervasive anxiety in this recent Futurum post. In the business world, a tentative cultural embrace of AI may be the underlying factor behind the supposedly unsupportive culture. Indeed, the survey found little year-to-year change in the percentage of respondents overall -- in both in-production and evaluating organizations -- reporting lack of institutional support (22%) and highlighting “difficulties in identifying appropriate business use cases” (20%).
The findings also suggest the very real possibility that future failure of some in-production AI apps to achieve bottom-line objectives may confirm lingering skepticisms in many organizations. When we consider that the bulk of AI use was reported to be in research and development -- cited by just under half of all respondents -- followed by IT, which was cited by just over one-third, it becomes plausible to infer that many workers in other business functions still regard AI primarily as a tool of technical professionals, not as a tool for making their jobs more satisfying and productive.
Widening usage in the face of stubborn constraints
Enterprises continue to adopt AI across a wide range of business functional areas.
In addition to R&D and IT uses, the latest O’Reilly survey found considerable adoption of AI across industries and geographies for customer service (reported by just under 30% of respondents), marketing/advertising/PR (around 20%), and operations/facilities/fleet management (around 20%). There is also fairly even distribution of AI adoption in other functional business areas, a finding that held constant from the previous year’s survey.
Growth in AI adoption was consistent across all industries, geographies, and business functions included in the survey. The survey ran for a few weeks in December 2019 and generated 1,388 responses. Almost three-quarters of respondents said they work with data in their jobs. More than 70% work in technology roles. Almost 30% identify as data scientists, data engineers, AIOps engineers, or as people who manage them. Executives represent about 26% of the respondents. Close to 50% of respondents work in North America, most of them in the US.
But that growing AI adoption continues to run up against a stubborn constraint: finding the right people with the right skills to staff the growing range of strategy, development, governance, and operations roles surrounding this technology in the enterprise. Respondents reported difficulties in hiring and retaining people with AI skills as a significant impediment to AI adoption in the enterprise, though, at 17% in this year’s survey, the percentage reporting this as a barrier is slightly down from the previous findings.
In terms of specific skills deficits, more respondents highlighted a shortage of business analysts skilled in understanding AI use cases, with 49% reporting this vs. 47% in the previous survey. Approximately the same percentage of respondents in this year’s survey as in last year’s (58% this year vs. 57% last year) cited a lack of AI modeling and data science expertise as an impediment to adoption. The same applies to the other roles needed to build, manage, and optimize AI in production environments, with nearly 40% of respondents identifying AI data engineering as a discipline for which skills are lacking, and just under 25% reporting a lack of AI compute infrastructure skills.
Maturity with a deepening risk profile
Enterprises that adopt AI in production are adopting more mature practices, though these are still evolving.
One indicator of maturity is the degree to which AI-using organizations have instituted strong governance over the data and models used in these applications. However, the latest O’Reilly survey findings show that few organizations (only slight more than 20%) are using formal data governance controls -- e.g, data provenance, data lineage, and metadata management -- to support their in-production AI efforts. Nevertheless, more than 26% of respondents say their organizations plan to institute formal data governance processes and/or tools by next year, and nearly 35% expect to do within the next three years. However, there were no findings related to the adoption of formal governance controls on machine learning, deep learning, and other statistical models used in AI apps.
Another aspect of maturity is use of established practices for mitigating the risks associated with usage of AI in everyday business operations. When asked about the risks of deploying AI in the business, all respondents -- in-production and otherwise-- singled out “unexpected outcomes/predictions” as paramount. Though the study’s authors aren’t clear on this, my sense is that we’re to interpret this as AI that has run amok and has started to drive misguided and otherwise suboptimal decision support and automation scenarios. To a lesser extent, all respondents also mentioned a grab bag of AI-associated risks that includes bias, degradation, interpretability, transparency, privacy, security, reliability, and reproducibility.
Takeaway
Growth in enterprise AI adoption doesn’t necessarily imply that maturity of any specific organization’s deployment.
In this regard, I take issue with O’Reilly’s notion that an organization becomes a “mature” adopter of AI technologies simply by using them “for analysis or in production.” This glosses over the many nitty-gritty aspects of a sustainable IT management capability -- such as DevOps workflows, role definitions, infrastructure, and tooling -- that must be in place in an organization to qualify as truly mature.
Nevertheless, it’s increasingly clear that a mature AI practice must mitigate the risks with well-orchestrated practices that span teams throughout the AI modeling DevOps lifecycle. The survey results consistently show, from last year to this, that in-production enterprise AI practices address -- or, as the question phrases it, “check for during ML model building and deployment” -- many core risks. The key findings from the latest survey in this regard are:
About 55% of respondents check for interpretability and transparency of AI models
Around 48% stated that they’re checking for fairness and bias during model building and deployment
Around 46% of in-production AI practitioners check for predictive degradation or decay of deployed models
About 44% are attempting to ensure reproducibility of deployed models
Bear in mind that the survey doesn’t audit whether the respondents in fact are effectively managing the risks that they’re checking for. In fact, these are difficult metrics to manage in the complex AI DevOps lifecycle.
For further insights into these challenges, check out these articles I’ve published on AI modeling interpretability and transparency, fairness and bias, predictive degradation or decay, and reproducibility.
About the Author
You May Also Like