Establish AI Governance, Not Best Intentions, to Keep Companies HonestEstablish AI Governance, Not Best Intentions, to Keep Companies Honest
AI governance is a board-level responsibility to mitigate pressures from regulators and advocacy groups.
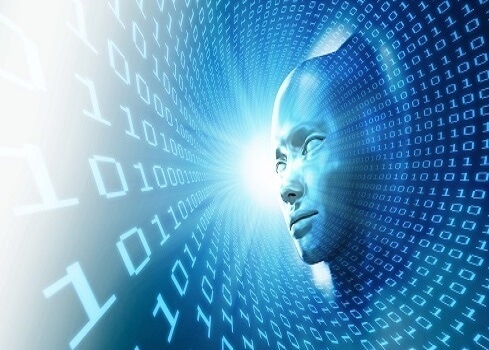
IBM, Microsoft and Amazon all recently announced they are either halting or pausing facial recognition technology initiatives. IBM even launched the Notre Dame-IBM Tech Ethics Lab, “a ‘convening sandbox’ for affiliated scholars and industry leaders to explore and evaluate ethical frameworks and ideas.” In my view, the governance that will yield ethical artificial intelligence (AI) -- specifically, unbiased decisioning based on AI -- won’t spring from an academic sandbox. AI governance is a board-level issue.
Boards of directors should care about AI governance because AI technology makes decisions that profoundly affect everyone. Will a borrower be invisibly discriminated against and denied a loan? Will a patient’s disease be incorrectly diagnosed? Will a citizen be unjustly arrested for a crime he did not commit? The increasing magnitude of AI’s life-altering decisions underscores the urgency with which AI fairness and bias should be ushered onto Boards’ agendas.
But AI governance is not about boards listening to guest scholars, or their own technology executives claiming they will “do no evil.” To eliminate bias, boards must understand and enforce auditable, immutable AI model governance based on four classic tenets of corporate governance: accountability, fairness, transparency, and responsibility.
Accountability is achieved only when each decision that occurs during the model development process -- down to an infinitesimal level -- is recorded in a way that cannot be altered or destroyed. Blockchain technology provides such a way to immutably capture each development decision and, equally important, who made it, who tested it, and who approved it.
Furthermore, AI models must be built according to a single company-wide model development covenant, with established bias testing and stability standards. A solid development framework further ensures that efforts don’t go to waste. I routinely sit with bank executives who tell me that 90% of their analytic models never make it into production due to the inexplicable “artistry” of unidentifiable data scientists.
Fairness requires that neither the model, nor the data it consumes, be biased. For example, facial recognition technology is well-known for inaccurately identifying women of color, due in part to the fact that AI developers are overwhelmingly white. Case in point: a 2018 study of facial-analysis software by MIT and Stanford University that found an identification error rate of 0.8% for light-skinned men and 34.7% for dark-skinned women.
To produce unbiased decisions, AI governance standards must impose AI model architectures that expose latent features. These are hidden relationships between data inputs that unexpectedly drive model performance, as derived from the model’s training data.
For example, if an AI model includes the brand and version of an individual’s mobile phone, machine learning algorithms activated during model training could combine these data to determine an ability to afford an expensive phone -- a characteristic that can impute income and, in turn, bias to other decisions, such as how much money a customer may borrow, at what rate.
A rigorous, and rigorously enforced, AI model development governance process, coupled with visibility into latent features, is a big part of how boards can enforce fairness by reducing bias.
Transparency is necessary to adapt analytic models to rapidly changing environments without introducing bias. The pandemic’s seesawing epidemiologic and economic conditions are a textbook example. Without an auditable, immutable system of record, companies have to either guess or pray that their AI models still perform accurately.
This is of critical importance as, say, credit card holders request credit limit increases to weather unemployment. Lenders want to extend as much additional credit as prudently possible, but to do so, they must feel secure that the models assisting such decisions can still be trusted.
Instead of ferreting through emails and directories or hunting down the data scientist who built the model, the bank’s existing staff can quickly consult an immutable system of record that documents all model tests, development decisions and outcomes. They can see what the credit origination model is sensitive to, determine if features are now becoming biased in the COVID environment, and build mitigation strategies based on the model’s audit investigation.
Responsibility is a heavy mantle to bear, but our societal climate underscores the need for companies to use AI technology with deep sensitivity to its impact. Analogous to the consumer mistrust that years of corporate data breaches have sown, AI blunders persist, to the world’s great detriment.
The future is clear, and it isn’t pretty: Boards of directors that fail to embrace their responsibility to deliver safe and unbiased AI will be battered by regulation, a cornucopia of litigation and powerful AI advocacy groups.
As a concerned citizen, I applaud boards’ waking up, and stepping up, to recognize the danger of unconstrained use of artificial intelligence. As a data scientist, I know that board oversight and government regulation of AI is necessary. Governance, not best intentions, is what keeps companies honest.
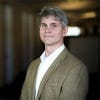
Scott Zoldi is Chief Analytics Officer of FICO, predictive analytics and decision management software company. He has authored 110 analytic patents with 56 patents granted and 54 pending.
About the Author
You May Also Like