Ethics, Privacy Issues Highlight Strata Data ConferenceEthics, Privacy Issues Highlight Strata Data Conference
Machine learning, artificial intelligence, and unified data platforms are the focus for data scientists attending the Strata Data Conference in New York.
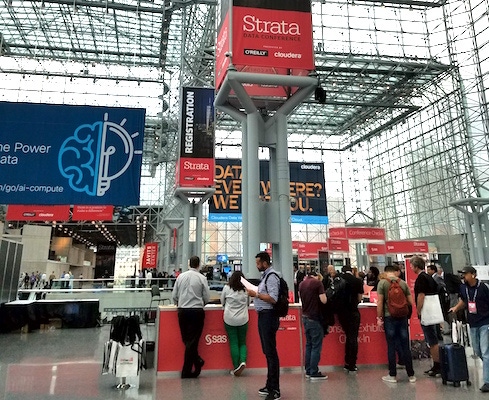
Machine learning and other artificial intelligence technologies took center stage at the Strata Data Conference in New York this week, exhibiting just how much the event has matured from its roots as a Hadoop-centered event. The changes, reflected in a name change for the event last year, are part of a larger overall shift and maturation in organizational adoption of a whole range of new technologies for improving insights that can drive competitive advantage.
Those technologies include a raft of open source offerings for data collection, management, storage, analytics, and streaming, and collected to assemble comprehensive platforms offered by companies previously known as Hadoop providers, such as Cloudera, Hortonworks, and MapR. Databricks is another big one that began as a Spark provider but now offers a stack of technologies, too.
This year's Strata Data Conference offered plenty of technical sessions, but also provided a big picture look at some of the larger issues facing the space -- diversity, data bias, and privacy, for instance. Here are some of the major trends captured in the first day of keynotes and presentations.
Fairness and avoiding bias in algorithms
From keynotes to individual sessions, fairness and avoiding bias in algorithms underpinned much of the content presented. While fairness and avoiding bias in AI seem like a noble goal in themselves, there are also business and financial reasons for protecting the integrity of your algorithms.
For instance, if you train your product recommendation engine with data that only comes from a narrow demographic, your algorithm will be less effective for a diverse audience. You could ultimately see lower sales.
The same principle applies to hiring a diverse team of data scientists, a theme discussed during the Women in Big Data lunch at the conference this week. A diverse team can provide protection against cultural biases that can creep into algorithm development and sabotage recommendation engines and other projects.
"We can improve our AI algorithms to filter out a candidate's demographic information, said Ziya Ma, vice president of the software and services group at Intel, which together with SAP sponsored the lunch. Ma noted that it was possible to filter out university, gender, and age, before viewing a list of qualified applicants to a job.
Differential Privacy
The General Data Protection Regulation went into effect in May 2018 in the European Union, causing organizations around the world to reevaluate their privacy policies. Even organizations in the US are feeling greater scrutiny on their privacy practices when it comes to consumer data after some high profile data leaks like the one at Facebook. This is a big issue now for enterprise organizations that need large data sets to train machine learning algorithms. If less consumer data is available, will organizations be able to adequately train their algorithms?
Differential privacy may be one answer. It works by protecting confidential data at the same time it offers an aggregate data product to data organizations. O'Reilly Chief Data Scientist Ben Lorica highlighted the idea of differential privacy as a potential solution during a keynote address yesterday at Strata, and a number of other sessions offered deeper details.
The shift in platforms, architecture
MapR Chief Application Architect Ted Dunning pointed out that data toolmakers need to evolve to offer an open platform that can meet the needs of all organizations -- something like Kubernetes for data harvesting, storage and analytics. That is something that all of the companies formerly known as Hadoop distributors are working to do now -- Cloudera, Databricks, Hortonworks, and MapR. Goldman Sachs Chief Data Officer Jeff Wecker, a former hedge fund manager, said during a keynote presentation that the industry has not yet realized the potential of tools such as machine learning, natural language processing, and other types of AI, as we march to a future where we will be collecting zettabytes of data.
"The next few years will be about applying all these emerging tools," Wecker said. "We've only begun to scratch the surface of applying these tools."
Transparency
Several presenters mentioned the need to be able to go back and demonstrate how machine learning algorithms reached their conclusions -- to provide a look into the "black box." For instance, Wecker said that "explainability" is necessary. Organizations may begin looking to tools that perform AI on AI. Such tools will be important not only for regulatory and compliance purposes, but they will also provide a greater level of confidence in the algorithms themselves as they are scrutinized for bias.
About the Author
You May Also Like