Go Big or Go Home: The CDO/CDAO’s Guide to AI LeadershipGo Big or Go Home: The CDO/CDAO’s Guide to AI Leadership
For IT professionals who assume leadership of their company’s fledgling AI practice, starting small with a “safe” project is not the path to AI success.
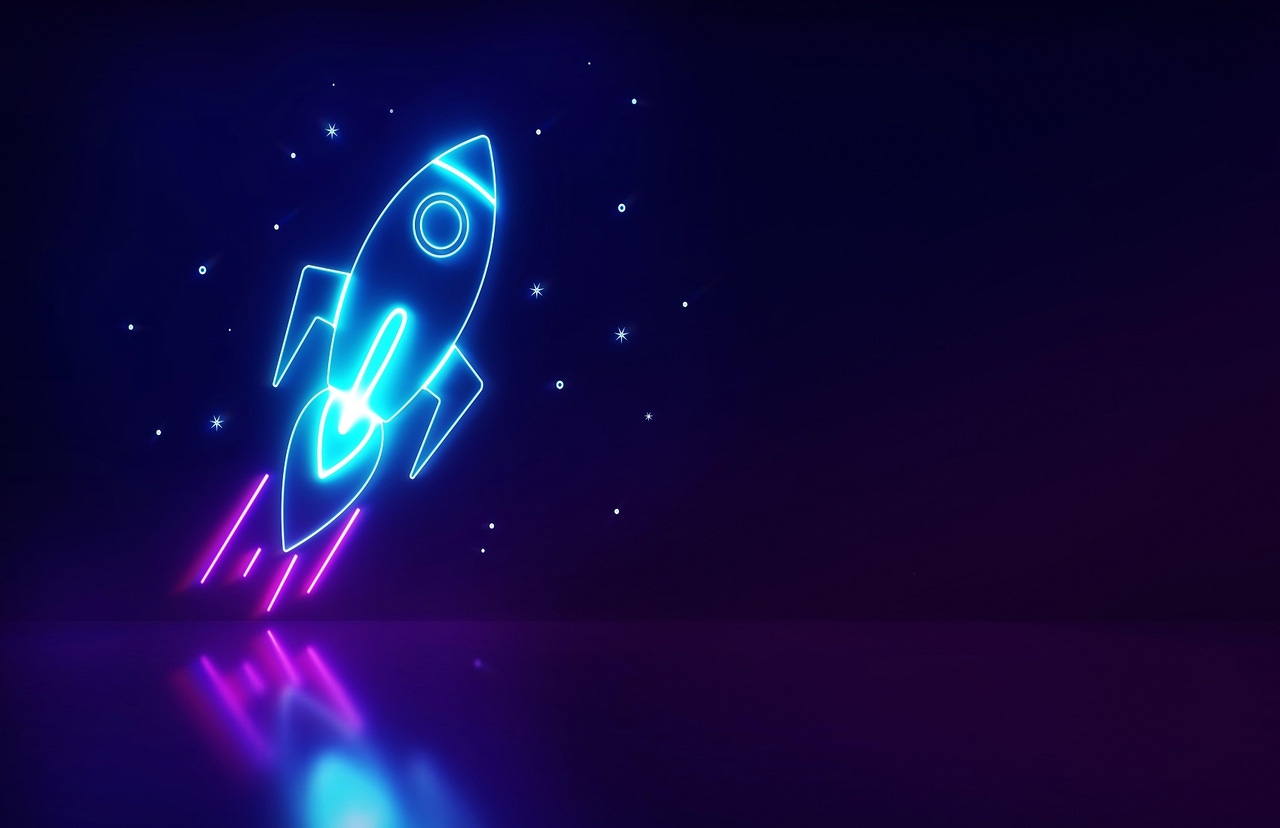
CEOs are facing mounting pressure to deploy game-changing enterprise artificial intelligence strategies. They are relying on their chief data officers (CDO) or chief data analytics officers (CDAO) to devise strategies for both predictive and generative AI (from this point on referred to simply as “AI”) that will move the needle on their company’s bottom line.
CDOs and CDAOs add tremendous business value through analytics, data governance, security and other vital functions, but for many, expanding their roles to include AI innovation requires adopting a challenging mind shift. If that situation sounds familiar, here’s a message you should heed: Go big, or go home.
Now is not the time to simply dip your company’s toe in the AI waters. A small, “safe” AI pilot project will not provide meaningful results. Instead, you must make big bets on AI, be willing to fail fast in your initial AI forays and learn from your missteps to achieve the ROI your CEO expects. While this may seem daunting, the right approach can unleash AI transformation.
New Vision and a New Way of Thinking
Few organizations have chief artificial intelligence officers (CAIO) with deep experience developing and executing comprehensive, meaningful AI strategies. Instead, CEOs are charging their CDO or CDAO to lead the organization’s AI initiatives. The mandate is often an aggressive target -- McKinsey estimates AI may increase productivity by upwards of 40% -- paired with a vague offer of support to get it done.
For the newly elevated CAIO, this can mean shifting from a conservative risk reduction approach to formulating a bold new vision for the enterprise -- a “wouldn't it be amazing if...” mindset that may not have a safe and secure path to a positive outcome.
4-Step Framework for an AI Transformation Mindset
The following steps comprise a systematic approach to pursuing meaningful AI initiatives for your organization:
1. Align with business objectives
Your company’s data is its greatest asset and the key to adapting AI to meet your business goals. Align your AI investments with a deep understanding of these goals, leveraging the richness of this proprietary data.
Encourage cross-functional collaboration to maximize both your domain expertise and proprietary data. Identify leaders who can provide insights into specific business functions and nuances in your company’s data, ensuring that AI initiatives are tailor-made for the unique challenges and opportunities within the enterprise.
2. Prioritize high impact use cases
Target AI opportunities with potential for the biggest payoffs. Quantify the impact of each use case, considering the unique insights that can be derived from your proprietary data. This approach enhances the accuracy of impact assessments and informs strategic decision-making. Establish a robust human-in-the-loop approval process early in the project, fostering transparency and ensuring alignment with organizational goals and regulatory requirements.
3. Build in risk assessment and mitigation
Recognize the importance of safeguarding proprietary data. Conduct comprehensive risk assessments, including considerations for data security, privacy, and ethical implications. Then, implement robust strategies based on your assessment. Prioritize data governance and compliance with industry and government regulations. Integrate regular compliance checks throughout the project, ensuring that all policies are adhered to at every stage.
4. Require continuous evaluation and adaptation
Define KPIs that reflect your business goals and create benchmarks to assess how your models and techniques perform. Regularly evaluate projects against these KPIs, using benchmarks derived from your company’s proprietary data to evaluate new innovative techniques.
There are multiple methods to leverage AI to achieve your business objectives. Embrace an iterative approach. Start with Retrieval Augmented Generation (RAG) with pre-built large language models (LLMs) but be prepared to fine-tune foundation models to adapt to the specific nuances of the enterprise's proprietary data.
Smaller models tailored to your domain, fine-tuned on your proprietary data, can often outperform larger models and are less expensive to operate. However, pre-built larger models are easier to use initially to determine if your chosen project is plausible, so don’t over-optimize too early.
Don’t Be Afraid to Make Big Bets on AI
Choosing the right AI projects is akin to pursuing a personal wealth investing strategy in a dynamic market. Rather than chasing the latest trends, organizations should focus on initiatives that align with long-term business objectives. At the same time, settling for conservative investments that offer both low risk and a low rate of return is not the path to true prosperity. Nor will it enable your business to gain a competitive advantage.
The key to success is continuous iteration, continuous learning, and continuous collaboration. Enterprises that build agility into their AI operations by experimenting with fine-tuned models -- analyzing the shortcomings of their outputs, making all learnings available across the enterprise, and keeping the executive leadership team apprised of both progress and setbacks -- are more likely to see their AI big bets pay off handsomely.
About the Author
You May Also Like