How AI Drives Results for Data-Mature OrganizationsHow AI Drives Results for Data-Mature Organizations
AI efficiencies are dependent on data quality. Here are some best practices data-mature organizations can implement now.
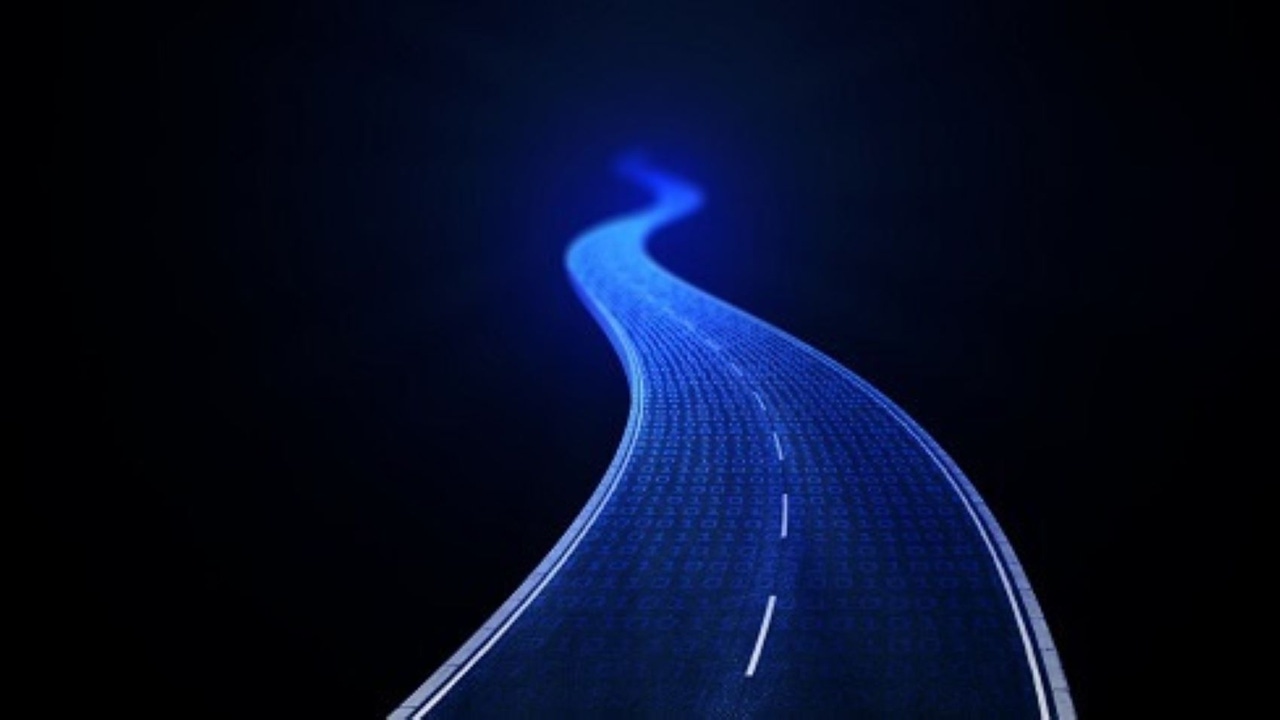
Every organization wants to optimize operational processes while keeping costs low. That’s why artificial intelligence has exploded onto the global stage in recent years. Companies see the promise of powerful automation tools, data suggestion and response systems, and the generative capabilities of platforms like OpenAI’s ChatGPT, Google’s Gemini, or Anthropic’s Claude and want to add them to their ever-growing toolbelt.
In the construction industry, we’re seeing clients lean on AI tools to summarize and track punch list items as they complete projects, brainstorm ideas for request for information (RFI) drafts, and other review and analytical tasks that significantly speed up the process from initial design to final build. AI is an immense boon, and I recommend that any company looking to improve the quality of its offerings implement AI where it can.
However, I also ask these organizations a simple question: “Will the data you have right now provide the results you’re looking for?”
Improving data quality isn’t as flashy as choosing the latest large language model, but it’s critical to AI’s efficacy -- ensuring that the information you get is helpful for your business and customers. It requires a foundational shift in how your business treats its data, from understanding the link between data and results to internalizing that information and transforming your business with data-centric policies and processes in mind.
How Data Quality Affects AI Results
The quality of the data you use determines the output you get from AI models. Without high-quality data, you’re effectively causing your business to leave productivity gains (and potential profits) on the table. Even the most powerful AI algorithms can only do so much if your data is inaccurate or inconsistent. Twenty-five percent of the highest-quality data isn’t available for public use so, to ensure the best possible results, it is essential to prioritize quality organization-specific data when training AI models.
When investigating the quality of your organization’s data, keep an eye out for the following critical flaws that can lead to poor AI results:
Incomplete data. Records lack critical information, or spreadsheets are missing data values throughout. If this data doesn’t exist, your analytical tools won’t have the comprehensive insight they need to provide actionable results.
Inconsistent data. Different regional methods for calculating and storing data may create incompatibilities when collating data into a single repository. These misalignments can lead to confusion in data processing and contribute to errors in output.
Duplicate data. Multiple databases exist to store the same information, creating data clutter that is difficult to sift through. Not only does this increase the storage required to maintain this data, but it also raises the cost of processing it through AI, significantly reducing operational efficiency.
Delays in data production. Inefficient processes increase the lead time between data gathering, cleaning, and usage, making the information you have obsolete before you can leverage it to benefit your business.
How Data-Mature Organizations Approach Data Quality
Once you understand what the potential flaws in your data might look like, you can correct them. In my experience, organizations that address the root causes of their data quality problems are poised to get the most out of AI integrations. The most successful of them share four common characteristics:
1. They view their data as an asset
Analysts expect global data storage to reach 200 zettabytes by 2025. Every organization will store and process data as a part of day-to-day operations. However, the ones that get the best results from their AI models understand that data isn’t just something that takes up digital space -- it’s an asset to be grown and cultivated with a steady hand.
That means managing data isn’t just a problem for your IT department to deal with. It needs buy-in from key stakeholders, preferably by building it into your organization’s structure at the executive level. Doing so will help you develop more effective solutions tailored to your organization’s unique needs. It will also help you leverage this data throughout the business to improve your product offerings, up to and including any AI models you use.
Automated data collection is a critical means for maximizing the value of data. Manual input can be erroneous, time-consuming, and limited in scope. Most companies that need accurate information for decision making do this effectively by establishing as many guardrails as possible such as suggestions, likely values, third party information, and standard drop-down lists.
2. They build standardized processes
First-party data is a key market differentiator. When properly sourced and vetted, high-quality data can give you a competitive advantage with insight no other organization can access. As a result, your data needs to be gathered from trusted sources and processed uniformly so it’s accessible throughout the organization and secured against cyberattacks.
Standardized processes help organizations achieve these goals. They ensure consistency and accuracy regardless of how data is ingested, which department gathers it, or who uses it. They also help to break down silos and improve intra-organizational accessibility, which is crucial for developing a genuinely unified and holistic approach to data storage. Build these processes into data gathering, training, and usage protocols, and enforce them across the organization to see better results.
3. They install comprehensive data governance policies
AI models need to be trained on data to provide accurate results. However, this data can be exposed (whether unintentionally or through malicious activity) without effective data governance policies in place.
These policies dictate how data can be used, whether it can be exposed to AI models for training or excluded in part or in whole. They also help your models align with required industry and governmental security and privacy regulations. Improving and enforcing your organization’s data governance policies will enhance its security stance while improving the overall quality of its output.
4. They invest in employee training
Less than half of executives believe their leaders have the knowledge to use AI safely and effectively. Investing in training opportunities in data gathering and AI usage will only make your workforce more capable of getting better results from any tools at its disposal. Training also helps generate buy-in from employees who are skeptical about how AI can improve their workflow while assuaging fears about potential privacy or security roadblocks.
By overcoming these challenges, you’ll build a data-centric foundation for your organization. It won’t just help you navigate a rapidly shifting AI landscape; it’ll help you adapt to an increasingly digital-forward future while maintaining high-quality data, whatever comes on the horizon.
About the Author
You May Also Like