How Synthetic Data Can Help Train AI and Maintain PrivacyHow Synthetic Data Can Help Train AI and Maintain Privacy
IBM, Gartner, and Datavant discuss legitimate uses for fake data.
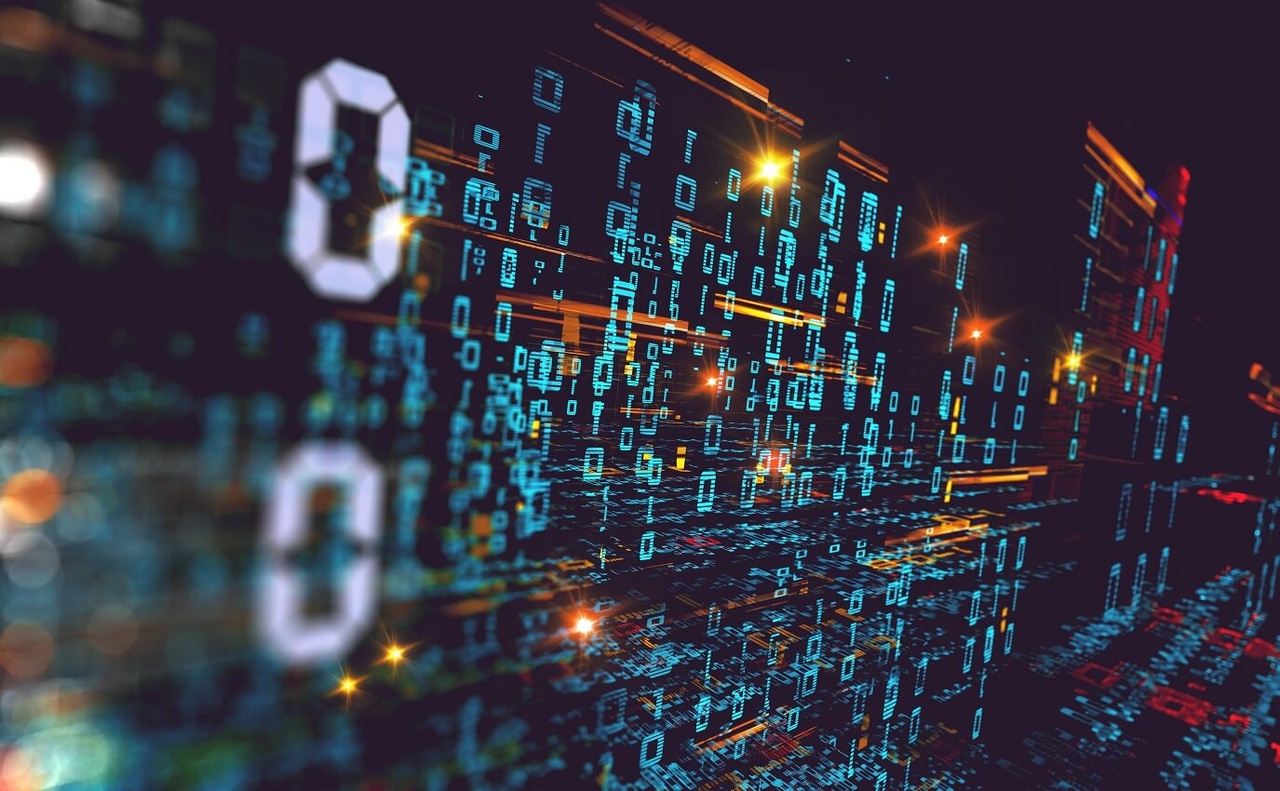
It is not always feasible, or ethical, to use live data to train AI or test out software platforms -- making a case for synthetic and augmented data to solve certain development needs. Stakeholders such as IBM, Gartner, and Datavant share some insights on benefits synthetic data can offer.
By using data generated by algorithms or through augmentation of real data, developers can put platforms and AI through their paces. This can address such needs as maintaining privacy, for example, if personal data cannot be released to a third party to test and develop software for financial transactions or the healthcare space. The use of synthetic data, in a world increasingly driven by data, is poised to continue to escalate.
Some might wonder if synthetic data can be abused to misinform or mislead others. For example, the purported use of synthetic and augmented data factors into a $175 million fraud case where the defendant is accused of paying a data scientist to cook up information for customers who did not exist, along with other acts.
Regardless of that case, synthetic data is often used legitimately and above board to develop and test AI and software systems with the likes of IBM working with it to train AI models.
A Reflection of Digital Realness
Synthetic data comes from an engine that uses real data to generate such output, says Jonah Leshin, head of privacy research for Datavant. Developers need essential properties in synthetic data, he says, that allow it to act as a facsimile of the original data. “It represents a copy that maintains the patterns from the original data so that when you use the synthetic data for analysis, the insights that you derive can speak to insights that are derivable from the original dataset,” Leshin says.
The development of a data pipeline, he says, may use synthetic data for analysis and when making entries into databases. “If you want to have some data to work with to kind of seed this workflow to kind of test it, synthetic data can be valuable for that,” he says, “if you're waiting on the real data.” There might also be a regulatory or privacy concerns that limit or prevent the use of real data. “Using it in that way is kind of almost like a dry run for the real data is of value,” Leshin says.
In instances where small datasets are not sufficient to work with, amplified data resources might do the trick. “You can use synthetic data to augment the original data by creating what we can think about as multiple copies of the original dataset,” he says. “It’s not multiple identical copies. It’s viewing their real dataset as if it were part of some larger population and viewing these synthetic data outputs as if they are random samples from that larger population.”
Where Synthetic Data Sees Use
Common use cases for synthetic data include software engineering when new features are built but no production data is available, says Jim Scheibmeir, senior director analyst with Gartner. For instance, if software is tested for an autonomous vehicle, and it needs new information about the weather or obstructions in the road, he says. Different scenarios can be generated to test that autonomous algorithm to prepare it.
Data scientists who are trying to create new algorithms, Scheibmeir says, or need to prove out new hypotheses might struggle to get their hands on production data. That limited availability might have to do with restricted access, compliance, or regulation, making synthetic data attractive.
The rise of generative AI might also play a role in synthetic data generation. “Certainly, ChatGPT is going to reinvigorate our imagination of what generative can do for us,” Scheibmeir says. “Gartner urges organizations to look at proper test data management, including synthetic data generation, for a few different reasons.” The growing move for data regulation and compliance with such laws as the European Union’s General Data Protection Regulation and the California Consumer Privacy Act could make data even harder to obtain. “There’s other states in the US that are picking up legislation, whether it’s Utah, Colorado, Virginia, or Connecticut,” Scheibmeir says.
Improving the experiences of developers is another reason for synthetic data’s growing use, he says. Unleashing a firehose of data at software engineers today might lead to significant cognitive overload, Scheibmeir says, when working with testing and test environments. “Another reason that we need to invest in test data management and synthetic data generation is to ease the burden of the engineers that we invest a lot in today,” he says.
Synthetic Data = Data Protection
IBM says it has been working with synthetic data as a means to test and train AI models by using augmented or replaced data to protect sensitive data and avoid bias. For example, synthetic data can be used to test stock prediction models for security flaws, IBM says, and see how stock prediction models that scour social media for tips respond to fake quote tweets.
Mitigating risk about transaction information is one of the reasons synthetic data is tapped, says Inkit Padhi, IBM Research engineer. For instance, if a third party is developing a resource that would eventually work with credit card transactions, the financial institution likely cannot share actual data because of the potential risks.
Synthetic data still requires controls and monitoring, Padhi says, to catch issues such as data leakage where private, personal data might seep into the mix. There is also a need, he says, to check synthetic data for fairness in what it presents. “If you generate synthetic data by mimicking exactly how real data is, that bias will be replicated to synthetic data,” Padhi says. “If the data has biased, the model that you train, the machine learning that you train will propagate these biases as well.”
What to Read Next:
Should There Be Enforceable Ethics Regulations on Generative AI?
What Just Broke?: Alleged Dark Deals in Synthetic Data
What Just Broke?: Digital Ethics in the Time of Generative AI
About the Author
You May Also Like