How to Avoid Common AI Newbie MistakesHow to Avoid Common AI Newbie Mistakes
To err is human, but AI doesn't really care about that. It's up to you to ensure that your first AI project doesn't turn into a big, costly mistake.
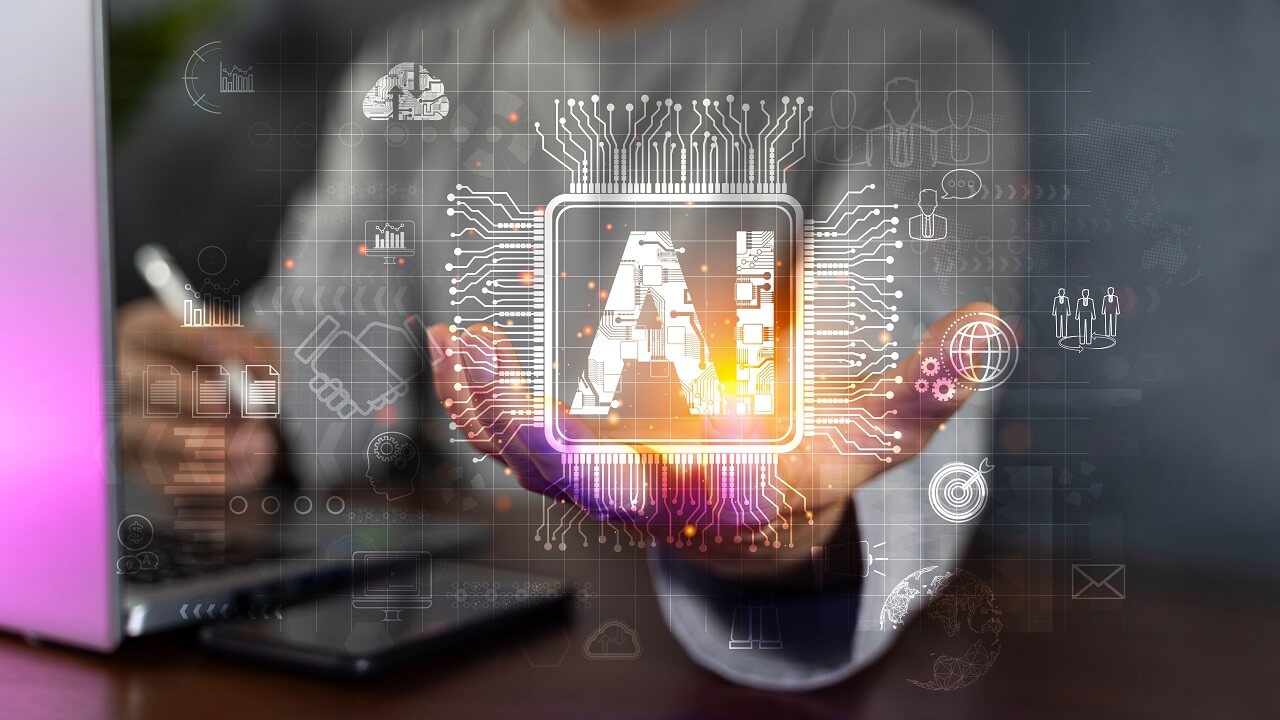
"Anyone who has never made a mistake has never tried anything new," Albert Einstein observed. When it comes to building your first AI project, you'll certainly have plenty of opportunities to learn from your mistakes.
Here's a rundown of the top five mistakes new AI adopters make, and how to avoid them.
1. Underestimating project complexity
New AI adopters often underestimate the complexity of integrating AI into their existing systems and processes, says Cristobal Fresno Rodriguez, business intelligence analyst at software development firm BairesDev. "[They] believe that AI can be a quick fix, or a plug-and-play solution, but the reality is far more intricate," he notes via email.
Underestimating AI's complexity generally results in projects that fail to deliver expected results. "This can lead to wasted resources, both in terms of time and money, and can create a sense of disillusionment with AI technologies within the organization," Rodriguez warns. "Additionally, this failure can erode trust in AI initiatives and make it more difficult to secure buy-in for future projects."
"It's crucial for organizations to approach AI adoption with a strategic and well-thought-out plan," Rodriguez advises.
2. Project indecision
Overzealous new AI adopters often make a false start by failing to fully define their project's intended purpose. "With the advent of generative AI, we've seen firms attempting to perform a number of proof of concepts, but they don't make it to prime time since the use case doesn't result in a business case," says Prasad Sankaran, executive vice president at IT services and consulting firm Cognizant, via email.
GenAI requires careful attention to trust, transparency, and security, and must be approached responsibly, Sankaran says. "This is essential for scaling AI and delivering value to customers." He believes that new AI adopters should embrace GenAI with a clear vision and strategy, as well as the appropriate guardrails, checks, and balances.
Decide what you want to do with GenAI and how you will measure success, Sankaran recommends. "Think about what data, tools, platforms, and skills you'll need, and how much money and time you can spend," he adds. "Make sure you follow the ethical and security standards for AI [and] use data and feedback to test and improve your AI solutions."
3. Believing that AI can replace humans
AI can mimic human cognitive functions, such as learning and problem-solving, but the key is to use the technology as a complement to the work that needs to be done, says Lauren Sallata, CMO with information management and digital services firm Ricoh North America. "Creating efficiency is a more realistic strategy than assuming AI will fully automate work," she notes via email.
When AI isn't adopted thoughtfully and carefully, organizations risk losing the potential benefit of optimizing specific use cases. "For example, building frameworks and processes around efficiency will help small teams increase their productivity while maintaining quality," Sallata says. "The best adoption plan is to make humans the centerpiece of your strategy."
4. Data neglection
A common mistake made by new AI adopters, particularly those looking to streamline and optimize financial operations, is underestimating the importance of high-quality, structured data. "Many companies believe that simply implementing AI solutions will solve any business problem," says Dan Drees, president of accounts payable software provider AvidXchange. "AI systems are only as good as the data they're trained on," he explains via email. "Without clean, relevant, and well-organized data, AI cannot perform accurately or effectively."
The typical result of poor-quality data is inaccurate predictions and insights. "When used effectively AI can, for example, help finance departments streamline their processes and increase efficiencies all while taking out additional steps such as manual tracking and continuous monitoring," Drees says. "If your systems aren't using quality data, it can lead to incorrect decisions, wasted resources, and ultimately poorer results, all of which can cost more time."
Drees recommends investing time and resources in data preparation before implementing an AI solution. "This includes cleaning and structuring the data, ensuring that it's relevant to the problems you want to solve, and continuously updating and maintaining the data to keep it accurate."
5. Stupid, useless projects
The biggest mistake many new AI adopters make is falling victim to "the shiny new toy syndrome," says Nikolaus Kimla, CEO of customer relationship management (CRM) software firm Pipeliner CRM. "They adopt AI tools because they sound great without properly assessing their applicability," he explains via email.
Adopting AI tools simply because they sound great leads to wasted time, Kimla warns. "Jumping from shiny new tool to shiny new tool negatively impacts productivity and means your team rarely has access to the solutions they need."
AI solutions should always align with business objectives, Kimla states. "AI should augment human capabilities and improve specific business processes rather than be adopted for novelty and show."
About the Author
You May Also Like