How to Build an Optimal Machine Learning TeamHow to Build an Optimal Machine Learning Team
An effective ML team is constantly evolving based on many different factors. Assess your specific needs and use cases before putting a team into action.
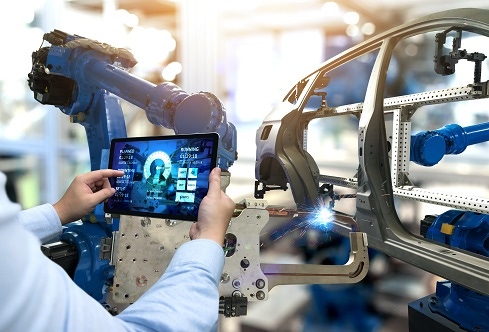
Machine learning solutions and workflows are meant to save time and vastly improve operational efficiency, but you still need the right human team to ensure every aspect is optimized and running on all cylinders.
Before getting started with finding the right people, you should take stock of the business problem at hand. The goal of an ML initiative may be to optimize rote business processes (e.g. automation) or it may be to establish a core piece business offering. No matter the case, it is imperative to first establish how the ML model fits within the greater workflow. Once your organization understands the implications of ML on the business, then it can begin to assemble the optimal team.
In an ideal world, what does that team look like? What mix of talent is needed to optimize results? The short answer is that it can vary based on your needs in a given instance. That said, in most cases, you won’t need to hire a full-stack ML team. On the most basic level, you should have one data scientist on board in an advisory role, whether it’s as a consultant or a member of the board, to help the engineering team figure out what it needs to do as challenges arise.
Starting with scientists and engineers, let’s take a closer look at the roles that every ML team is likely to utilize at one point or another:
Data scientists and ML engineers
Data scientists are the team members that might be able to create new architectures such as neural networks to solve the business problem because they’re constantly up to date on the latest technology. Their role is to guide the team on the state-of-the-art and how to structure the problem to achieve the appropriate business metrics. Scientists also often help engineers troubleshoot the ML model -- the papers they’ve read and conferences they’ve attended will likely inform them on which variations you can accomplish higher accuracy (or other metrics) with a specific model.
ML engineers are the folks who know which type of architecture they need to build and train the ML model. They should be well-versed in trying out different data, saving the model, and figuring out how to get the model into production. In addition, engineers help make sure the entire pipeline can support rapid development and iterative increments after launch. In short, model management is the responsibility of the engineering team.
For medium-sized companies, having more engineers than scientists is most often the way to go. However, if a workflow is at the proof-of-concept level, having more scientists available is preferable because they know how to solve the technological problems involved. If the said problem is already solved (for example, a large body of scientific literature or frameworks already exist), then multiple scientists likely won’t be necessary.
Once a workflow is launched and you want to iteratively improve the model, engineers will update the model to new data, while scientists help understand emerging improvements in the field and how to apply them. But keep in mind that some ML techniques like deep learning are not yet mature and are evolving at a break-neck pace. What team members learned last year might not be accurate or relevant now.
Development and operations (DevOps)
Organizations need DevOps and operations support to launch a model and manage the continuous delivery pipeline. They need to understand if the model is working as intended and they need to oversee and maintain the technology behind deploying the model. If an organization uses a cloud provider, however, they will most likely take care of scaling for you.
The bigger challenge is how to interface the product or engineering teams with the rest of the system. At this point you need a technical product manager role filled by someone who can lead those conversations and understand the requirements of the product side to figure out feasibility and, just as importantly, prevent the model creation from getting too research-oriented or too costly. Scientists or engineers may try to squeeze an extra percent of accuracy out of the model, and product managers need to figure out if you need to squeeze or not. Product managers also need technical acumen -- the ability to understand the workflow in terms of how it’s affecting the business case and how it’s making money.
Engineers can and often do interact with DevOps engineers, but you want to make sure the interface between the ML team and traditional departments is seamless. For example, maybe a mobile app is talking to the model, but it wasn’t previously. You need the right people in place to make sure the API is integrated and working.
You also need expertise in the deep learning space. A large part of a scientist’s time is spent in only one field because the speed at which deep learning is evolving requires them to specialize. Someone also must be focused on computer vision. After the initial foundation of understanding is in place, knowledge starts to diverge based on specific use cases. You need someone who knows how to get things out fast and iterate. Accuracy for video is very different than that for text, for example, depending on what the application is.
During the early stages when defining whether you can do an ML solution or not, it’s better to have an expert in house. As it stands, the consultancy market for ML isn’t very mature, so it would be hard to find someone to consult -- having a Ph.D. in-house is a much better situation. Your ML engineers might not be able to distinguish if there’s a unique case needed at the architecture level as opposed to an engineer who is more informed about “object detection.”
In the end, depending on strengths at your disposal, you need to decide if you need a scientist or ML engineer. The question you ask should be, “Has this problem been solved before?” if the answer is no, then a scientist is required. But if the answer is yes, then an engineer can use existing solutions and customize them. An effective ML team is constantly evolving based on many different factors, so it’s important to first assess your specific needs and use cases before putting a team into action.
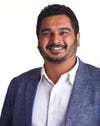
Rahul Parundekar is a Deep Learning and Artificial Intelligence Expert with 10+ years of experience in building AI products, research, and thought leadership. He is passionate about building AI solutions for improving the Human Experience. He believes that as AI becomes more commonplace, providing increasing levels of automation, we need to build intelligent systems that work together with humans and contribute to the larger human endeavor.
About the Author
You May Also Like