How to Operationalize Your Machine Learning ProjectsHow to Operationalize Your Machine Learning Projects
Operationalizing those data science, analytics, and machine learning projects is one of the top concerns of IT leaders. But the same tried-and-true best practices you've used for other IT projects can guide you on these new technologies, too.
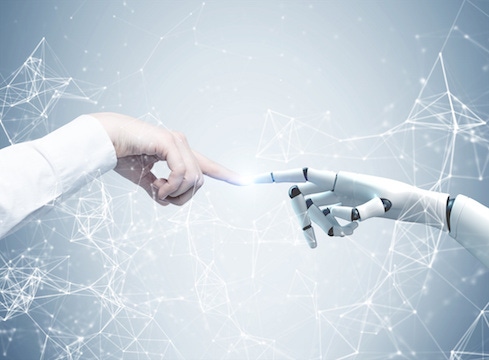
Everyone knows that to compete in the future, you need to invest in machine learning, artificial intelligence, data, and analytics. But there still can be a big gap between knowing that you need to do it and figuring out how to do it in a way that is meaningful for your business.
Putting these technologies into production systems continues to be a challenge for many enterprises, according to Erick Brethenoux, a research director at Gartner.
"Development is academic. Production is economics," Brethenoux said during the session Operationalizing Data Science and Machine Learning Initiatives, delivered at the Gartner Data and Analytics Summit in Orlando, Florida.
Yet many of the best practices for putting these technologies into production probably sound familiar. They are the same rules that Brethenoux has recommended for years, and that you have probably applied to your IT projects throughout your career.
For instance, Brethenoux recommends that you start by selecting use cases for the technologies. But don't just do this in a vacuum. Your use case research should start by talking to the stakeholders in the business. They will be a good source of information about what projects may be appropriate and what the KPIs (key performance indicators) should be to measure the performance of any project. Then select business owners and research data resources.
Once you have a list of potential use cases, the next step is to prioritize them. Put those use cases that would offer the greatest business value at the top of the list. Then look at a few other factors. Are there likely to be roadblocks for any of these projects? What are they? Also, estimate the technical complexity of each use case. Finally, determine whether each use case is "a business extender" or "a game changer."
Evaluate each of the possible use cases by these factors, and "stack rank" the use cases. Brethenoux recommends going with business-extender use cases first -- maybe three to six of them -- and then adding a few "game changers." Start with use cases that have lower technical complexity and those that have spinoff potential, Brethenoux said.
Once you have created your use case, there are two implementation cycles. First, once the model is published, you test the assumptions made about the results that you expected. Is the model delivering on the KPIs and business results that you expected?
"Is it possible that between the development phase and the deployment that some things have changed"" asked Brethenoux.
Then, the second cycle acts as the implementation phase. This is where your organization tests integration and validates KPIs.
Brethenoux recommends putting together a designated team to accomplish this -- a center of excellence or data science lab. This should include members with subject matter expertise, members with business domain expertise, and members with data science expertise.
You may also want to consider creating a steering committee for these projects. This group, made up of members from the general business, not IT, should be able to provide context on what other use cases the data science lab should be pursuing.
Brethenoux said that IT leaders should look for the talent for these teams internally. He has seen many job descriptions with so many requirements that "I'm thinking there are two people in the world who could take that job. You need to pare that down. Often the people you want are in your own organization. Solve for talent from within."
Read more of our coverage of AI in the enterprise here:
AI, Machine Learning, Data Science: What Enterprises Are Doing
Ford Motor IT's Changing Direction
About the Author
You May Also Like