Is AI Bias Artificial Intelligence’s Fatal Flaw?Is AI Bias Artificial Intelligence’s Fatal Flaw?
Research shows that AI frequently churns out biased results. Will it ever be possible to create fully objective algorithms?
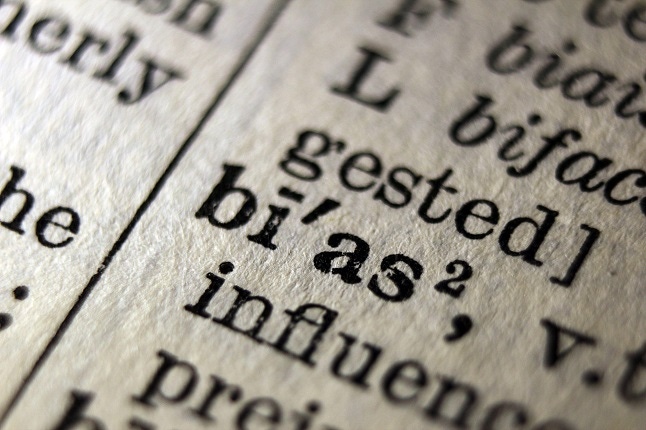
AI bias is a well-known and stubborn challenge. Bias typically occurs when algorithms are trained on data sets that are skewed or not fully representative of the groups they aim to serve. Over the past several years, researchers worldwide have struggled with the issue of how human biases impact AI systems with often harmful results.
Bias is probably the single most challenging problem for the future of artificial intelligence systems, states Arthur Maccabe, executive director of the Institute for Computation and Data-Enabled Insight at the University of Arizona. Bias is an observation and, as such, is itself not a problem, he says. The problem occurs when a biased system is used to make decisions.
AI can propagate social injustices when fed with biased training data, warns Michele Samorani, an associate professor of information systems and analytics at Santa Clara University’s Leavey School of Business. “Suppose I’m a university, and I want to train an AI system to screen college applications,” he says. “If I train that system with the admission decisions made in the past, then any biases that humans had in the past will be present in the AI.”
Unintended AI Bias Consequences
AI bias’s impact is far-reaching. “Biased AI systems can reinforce societal stereotypes, discriminate against certain groups, or perpetuate existing inequalities,” observes Alice Xiang, global head of AI ethics at Sony Group, and the lead AI ethics research scientist at Sony AI. “It can result in discriminatory practices in areas such as hiring, healthcare, or law enforcement.” Bias can also erode trust in technology and hinder AI adoption, she notes.
Addressing AI bias will require a multi-faceted approach, Xiang says. “It starts with diverse and representative training data that accurately reflects the real-world population,” she states. “It’s crucial to involve individuals from diverse backgrounds, including those who are affected by the AI system, in the decision-making process.”
Maccabe says that the data used to train AI systems must accurately represent all of society. “This is likely to be unattainable in general, so we must find ways to document the biases included in the training data and limit the use of AI systems trained on this data to contexts where these biases are not critical,” he advises. Comparing AI bias testing to human learning bias testing, Maccabe envisions the adoption of processes that ensure an AI system has been exposed to a valid training set or subjected to validity testing before certifying that it can be safely used.
While eliminating AI bias will be challenging, minimizing its impact is achievable through concerted efforts and technological advancements, says Beena Ammanath, executive director of the Deloitte AI Institute. She suggests that AI models should be subjected to rigorous testing, validation, and evaluation processes to identify biases and prevent long-term ill consequences. Ammanath observes that most AI adopters already have the tools and resources needed to address bias proactively through a holistic approach that includes education, a common language, and unrelenting awareness. “By embracing these measures, we can work toward a future where AI technologies are more equitable and unbiased,” she notes.
A Challenging Task
Permanently eradicating AI bias won’t be easy. To identify and mitigate bias and other AI ethics harms, it’s necessary to understand AI systems, how they could have unintended consequences, how they might be attacked or misused, and how they might harm people, Xiang says. “It’s challenging for development, engineering, product, and legal teams to solve all of these problems on their own, so recently there has been a rise in AI ethics teams at companies that incorporate AI into their products and services,” she explains. “These teams are focused on developing and implementing best practices in AI model development and training.”
One of the key challenges facing AI professionals is the need to establish data sets that are both large enough to train AI to do something useful, as well as representative of the context in which the AI will be used. “Creating a training data set that meets both objectives can be time-consuming and very expensive,” Maccabe warns.
In some instances, developers can settle for data sets that are simply “close enough,” Maccabe says. “The true magic of Google Translate was finding a data set that was good enough to train the system for its intended purpos: helping people who speak different languages find ways to communicate,” he notes. “The cost of creating this data set from scratch would likely have been prohibitive.”
Standards, Regulations, and Processes
Santa Clara University’s Samorani says there’s a growing need to create standards and regulations for auditing AI systems for bias. “I’m optimistic about this,” he states. “I think that with the right regulations and audit systems in place, we can reduce AI bias to a point it is no longer a concern.”
Xiang notes that efforts are already underway to address AI bias by implementing ethical data collection processes, developing more diverse training datasets, adopting fairness metrics and evaluation frameworks, and promoting transparency and accountability in the development and deployment of AI systems.
In the meantime, Ammanath believes that it’s important to continue educating organization stakeholders about AI risk bias, regardless of their technical and business responsibilities. “Organizations should prioritize educating their employees on company ethics as well as AI ethics principles,” she advises.
What to Read Next:
AI’s Equality Problem Needs Solution: Experts
Should There Be Enforceable Ethics Regulations on Generative AI?
About the Author
You May Also Like