Multimodal AI: The Future of Enterprise Intelligence?Multimodal AI: The Future of Enterprise Intelligence?
Multimodal artificial intelligence can serve as the eyes, ears, and brains behind generative AI. It will fundamentally change business and IT.
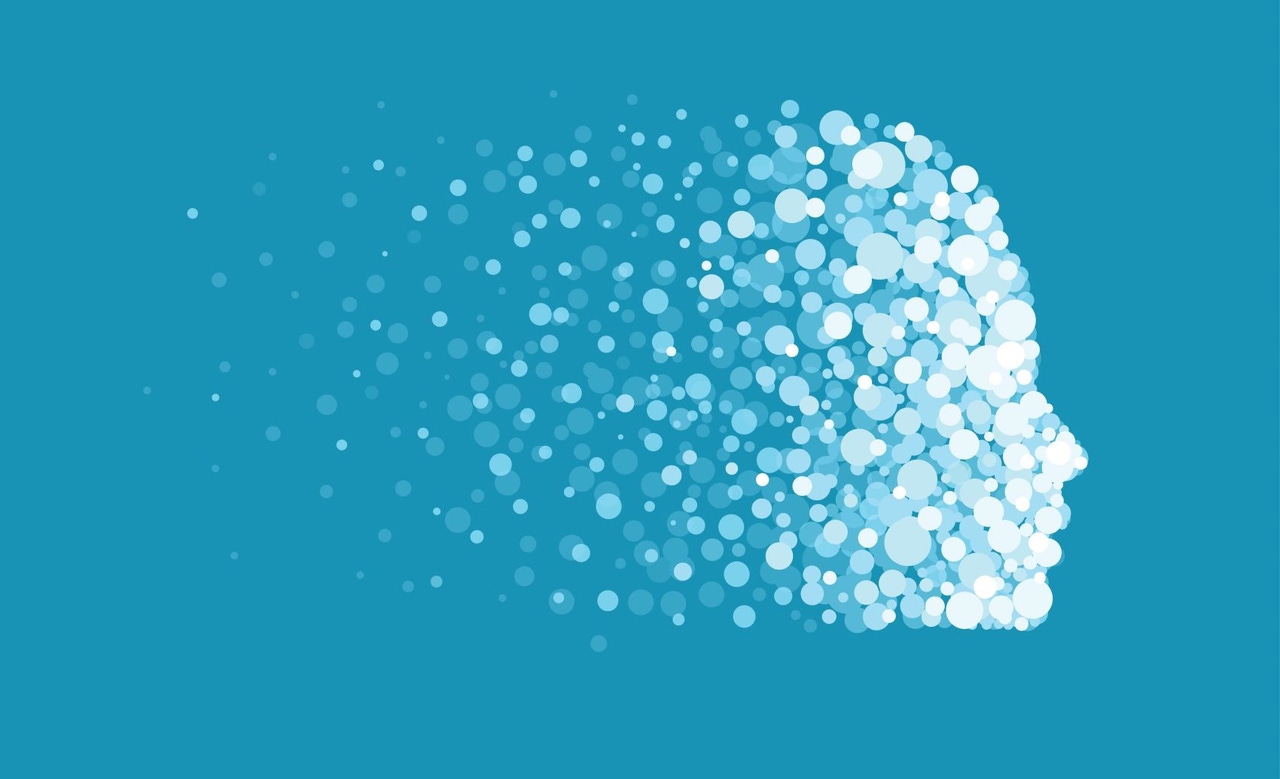
No technology in history has achieved an adoption curve that rivals generative AI (GenAI). Already, organizations use it for everything from chatbots and content creation to product design and software development. The technology boosts efficiency, trims costs, and unlocks innovation.
Yet for all the gains there’s still a good deal of pain. Too often, generative AI systems do not recognize basic facts and information that humans take for granted. For example, they might misinterpret or misclassify events and produce flawed output, struggle to generate desired content, or fall short on more complex tasks that require a combination of text, audio, and video.
That’s where multimodal AI enters the equation. “Multimodal AI models are trained with multiple types of data simultaneously, such as images, video, audio, and text. This enables them to create a shared data representation that improves performance for different tasks,” explains Arun Chandrasekaran, distinguished VP and analyst for artificial intelligence at Gartner.
Adds Scott Likens, US and global chief AI engineering officer at PwC: “Multimodal AI can tackle more complex challenges, create more personalized experiences, and help companies adapt more effectively. It’s about versatility and deeper insights, which are crucial to staying ahead.”
Multimodal AI potentially touches chatbots, data analytics, robotics, and numerous other areas. According to research conducted by Gartner, only about 1% of companies were using the technology in 2023 but the figure is projected to jump to 40% by 2027. The technology will have a “transformational” impact on the business world, Gartner reports. “It enables use cases that previously weren’t possible,” Chandrasekaran says.
AI Comes to its Senses
What makes multimodal AI so appealing -- and powerful -- is its ability for AI to act more like a human being because it understands the world better. “Traditional machine learning uses a specific training set to predict output,” states Matthew Kropp, a partner and managing director at Boston Consulting Group. “Later, you look for ways to adjust the weights in the model. Multimodal AI expands the training data in the pursuit of more realistic results.”
PwC’s Likens compares multimodal AI to the human ability to multitask. “You can ask a question via audio and receive a written response or submit an image and then ask questions about it. The interoperability between mediums is seamless. For business leaders, that means making smarter decisions faster. You’re not just looking at text or just an image; you’re seeing the whole picture,” he says.
The result is systems that are far better aligned to handle real world tasks -- and tools that create more personalized experiences and deeper insights. For example, a chatbot might handle both text and images. This makes it possible for a user to describe a problem in words but also upload a photo of a broken product. A multimodal AI system might also understand video content and seamlessly extract cues that provide context -- and answers.
The results can be impressive. Multimodal systems can introduce visual question-answering and even complex audio and video generation, Chandrasekaran explains. This includes creating AI podcasts and instructional materials. Organizations also are better equipped to tune into market and consumer sentiment through various types of data.
Over the next few years, the range of multimodal inputs will increase beyond text, images, and video, Chandrasekaran says. Systems are likely to incorporate a greater range of audio data, sensor and IoT data, log files, code snippets and more. This will boost the accuracy, contextual awareness, and overall utility of chatbots, robots, diagnostics systems, and predictive maintenance tools.
Evolving Beyond the Bot
Multimodal models come with a major caveat: Stringing together a mélange of unimodal data models is not the same as constructing a purpose-built multimodal framework. “Multimodal data must be aligned and integrated. It is more complex because it has varying degrees of quality and comes in different formats than unimodal data,” Chandrasekaran explains.
Specific tools that aid in building multimodal frameworks are evolving rapidly. Cloud platforms AWS, Google, and Azure have introduced multimodal features into their toolkits. Pre-trained models like OpenAI’s CLIP (Contrastive Language-Image Pretraining) and BERT (Bidirectional Encoder Representations from Transformers) have appeared. And multimodal libraries and tools like MMDet (Multimodal Detection) and Hugging Face Transformers tie together diverse data sets.
CIOs and IT teams must take a hands-on approach to multimodal AI. An effective framework must fit an organization’s specific data and objectives, and data must be clean and clearly labeled. There’s also a need to address business risks that include data bias, privacy concerns, fairness standards, copyright concerns, and overall data accuracy. This requires appropriate training and evaluation techniques like cross-validation and accuracy metrics.
"Because multimodal AI involves diverse inputs -- text, images, audio, and video -- maintaining consistent data quality is key,” Likens notes. “Privacy concerns are equally critical, because multimodal data can reveal unintended patterns.” It’s also critical to keep humans in the loop. “Investing in responsible AI from the start helps companies manage risks, build trust, and stay ahead of government regulations,” he argues.
For now, organizations can benefit by reviewing applications, tools, and partners, Kropp says. This includes using open-source models and tools that help lower the entry barrier and reduce risks associated with major IT commitments. “Matching the model and the vendor with your desired use case is important. Different combinations result in different and potentially better results,” he notes.
Structural changes may also be in order, Chandrasekaran says. Among his suggestions? “Educate your AI team on multimodality, including the benefits and risks. Break up AI technical silos by encouraging AI experts to work on projects outside their area of technical specialization, such as natural language processing and computer vision. Expose AI teams to vendors that focus on multimodal models as part of the overall education process.”
Make no mistake, multimodal AI will emerge as a powerful force over the coming years. It will allow organizations to push both classical and generative AI performance to a new level. Concludes Likens: “Multimodal AI can form a more complete picture than any single data source could manage. It can resolve problems with missing or noisy data and fill the gaps. The result is an ability to understand things in a more complete way.”
About the Author
You May Also Like