Soccer Wonks Learn Tough Big Data LessonSoccer Wonks Learn Tough Big Data Lesson
Recent gameday experiment shows that in sports and in business, even the most detailed big data analysis is worthless if the questions don't make sense.
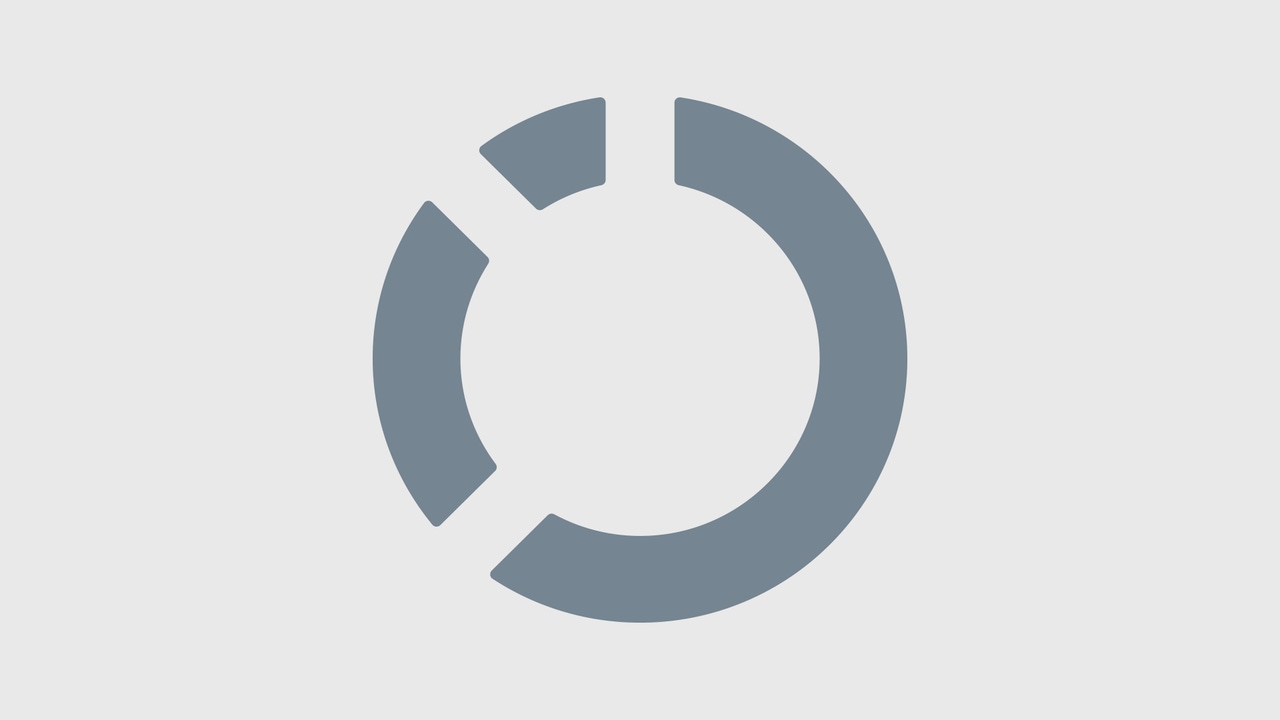
On July 25, a team of U.S.-based Major League Soccer players generated publicity in the United States and shock in Europe after beating Chelsea, a major power in the globally dominant British Premier League. But publicity and shock weren't all that the game produced.
Players from both teams generated thousands of individual data points on their positioning, conditioning, and performance--all collected by the miCoach GPS-based performance monitoring system from Adidas, which they wore during the game.
Gathering statistics on major-league play is nothing new; gathering statistics so detailed no human could ever perceive the actions they measure, let alone accurately record them by hand, is brand new. So is the expectation that the data will be pooled with as much other performance data as possible, then crunched using big data analytic techniques.
The result, theoretically, would give team managers the kind of information they need to assemble an unbeatable team, or train an existing team to exploit weaknesses in an opposing team that the opponent may not even be aware of.
It would be a big data miracle of the kind documented in Moneyball, the 2003 book describing how the Oakland Athletics built a strong roster despite a weak payroll budget by using statistics to identify players who would be unique assets to the team.
[ Learn how Big Data Could Fix School Shortcomings. ]
There is a good chance the data gleaned from the July 25 victory will make some U.S. teams better.
There's just as good a chance--according to sources with both practical and academic expertise on the topic--that non-statisticians will misunderstand the meaning of the results they see and make decisions reinforcing exactly the kind of performance they're trying to avoid.
Big Data Leads To Big Decisions, But Not Always The Right Ones
As it turns out, European professional soccer leagues have long been heavy users of statistical services from companies including Amisco, Opta, and Prozone--all of which were founded in the mid to late 1990s, long before Moneyball became a thing, according to Matt Aslett, research manager for The 451 Group.
Statistical analysis of players' performance, nutrition, physical condition, and other factors is far more intense than in the U.S., according to a BBC profile of Manchester City, which won the British Premier League championship in 2012 for the first time in 44 years.
Manchester City coaches--or their data-crunching counterparts--know the inner workings of their players so well they are able to concoct recovery drinks and nutritional supplements customized to the results of blood and saliva tests for each player. The supplements are given to players on their return from a hard practice so the team can be certain each player gets the right mixture of biochemical raw materials to repair the damage done by 90 minutes of running hard to capture a ball without touching it with their hands.
Much of the information--about injuries, fitness, and potential therapies, if not the biochemical profiles--is even published as part of the teams' effort to help keep fans up to date with minute changes in their favorite players' conditions.
Among other things, many teams attach GPS and vital-sign monitors to players' attire during practice to collect data on heart rate, stress load, distance covered, rate of acceleration and deceleration, and 100 other bits of data defining some qualities of their play.
Some statistics that seem critical are actually meaningless, however, Aslett wrote, while seemingly irrelevant numbers are crucial.
For example, there is virtually no correlation between the distance a player covers on the field during a game and the outcome. There is also little correlation between the number of tackles, shots on goal, or other specific on-field feats and the score at the end of the game.
On the other hand, according to a Financial Times interview with Manchester City performance analysis chief Gavin Fleig, using statistical analysis to predict where the ball or the other team's players will be can be a huge advantage.
"We would be looking at, 'If a defender cleared the ball from a long throw, where would the ball land? Well, this is the area it most commonly lands. Right, well that's where we'll put our man,'" Fleig told the Financial Times. Correlation Does Not Equal Causation
Rival Chelsea has collected more than 32 million data points over the course of 12,000 to 13,000 games, according to Mike Forde, the team's performance director, in the same Financial Times article.
It took time for team managers and their statistics gurus to understand which of those data points to pay attention to. The percentage of completed passes compared to the number of interceptions is a good indication of consistent victory, as is an error rate lower than 18%.
Traditional metrics such as the number of tackles, on the other hand, can lead teams to ignore potentially invaluable players, however, according to Soccernomics, the European-football version of Moneyball.
For example, retired team Milan star Paolo Maldini, who Aslett describes as "arguably one of the greatest defenders the world has ever seen," rarely made more than one tackle every other game. Viewed only by that statistic, he would probably be out of a job.
Judged according to details of his positioning, movement, and comparisons with players whose advance he was trying to prevent, it became obvious "he positioned himself so well he didn't need to tackle," according to Soccernomics.
That particular error was due to the tendency to measure the performance of individuals even while they engage in a team activity--corporate project teams, for example, as well as soccer.
Analyzing the results of such out-of-context metrics shows that judging players as stars according to their individual accomplishments could very well hurt the performance of the whole team by ignoring those whose team play makes the whole group successful, according to a study published in 2010 by Jordi Duch, Joshua S. Waitzman, and Luis A. Nunes Amaral, researchers at Northwestern University.
"Whereas there are contexts in which simple measures or statistics may provide a very complete picture of an individual's performance--think of golf, baseball, or a track event--for most situations of interest, objectively quantifying individual performances or individual contributions to team performance is far from trivial," the authors wrote.
"In the context of a soccer, where quantification has always been challenging, we are able to demonstrate that flow centrality provides a powerful objective quantification of individual and team performance," they concluded.
In other words: examining, highlighting, and rewarding players (or employees) according to their individual accomplishments can be anything from ineffective to counterproductive in situations in which the goal can only be accomplished with contributions from many players.
While the Northwestern researchers could not extrapolate their results into specific guidelines on how corporate managers could encourage more effective team play by their own employees, they did suggest the result should also apply to situations other than soccer.
The value of teamwork and team building is widely accepted within business-management circles, though it is unclear how many incentive systems genuinely reward teamwork at the expense of individual accomplishment.
Studies describing the value of teamwork and techniques to encourage it are mostly anecdotal and advisory, rather than quantitative, so it's difficult to extrapolate from them. However, it's easy to extrapolate how big data analytics can be misunderstood or misused by people trying to extract lessons from it.
Garbage In, Garbage Out
Using big data analytics to identify points of inefficiency, gaps in automation, and other elements in a specific business process may also affect an entire business, according to Bill Franks, chief analytics officer of global alliance programs at Teradata.
Corporate processes tend to depend on one another, not exist independently. So improving one process using data on only that process is just as likely to cause glitches in related activities and gum up the whole works, Franks wrote, as it is to improve things overall.
Even doing all the analytics correctly and improving systems in a coordinated way won't do much to improve a company that didn't pay as much attention to the quality of data going into a big data system as it does to the answers coming out, according to Steve Sarsfield of big data analytics vendor Talend.
Even something as simple as having one call center operator who occasionally misspells Main St. as "Mian St." will reduce the quality of the data overall and make it harder to depend on the kind of personalized, location-dependent recommendations a big data system might deliver for a single customer who seems to live part of the time on Main St. and part of the time in a location the map-search engine can't identify.
Even with top-quality data and analytics, making the results available to a marketing staff (for example) with too little training in statistics can cause those too enthusiastic about the results to adjust tactics every time they see a change on their dashboards "and end up changing direction so often that they lose sight of their goals," according to an article titled "Marketers Flunk the Big Data Test" in the Harvard Business Review.
The lesson seems to be that big data, no matter how great the potential or how ambitious the goal, can't point a team or a corporate staff in the right direction if there are flaws in the quality of the data, the questions, or how the answers are eventually used.
Without those three, no matter how big the data or how sophisticated the analytics, it may be more accurate to just guess the answer, rather than extracting the wrong one using advanced techniques and expensive technology no one quite understands how to use.
Attend Online Marketing Summit 2012 and gather the insights and strategies you need to make the right online marketing choices to deliver the most value for your business. The summit--in Santa Clara, Calif., Oct 22-25--offers four days of inspiration, connections, and practical learning. Attend Online Marketing Summit 2012 using code QJBQSA01 and receive a 25% discount on conference passes or a Free Expo Pass.
About the Author
You May Also Like