The Best Way to Get Started with Data AnalyticsThe Best Way to Get Started with Data Analytics
As data analytics matures, it's attracting a wider range of adopters. Here's how your organization can tap into this essential business technology.
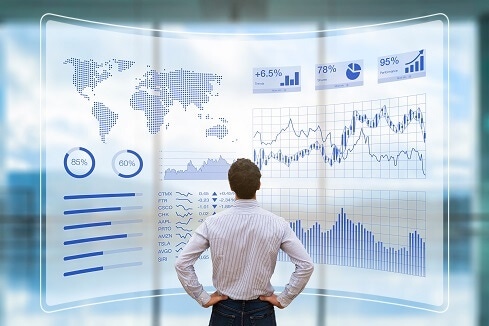
Over the past several years, enterprises of all types and sizes have transformed themselves into data-driven organizations. With the assistance of data analytics, it’s now possible to put customer and market data to work and obtain rewarding insights almost immediately.
While data analytics' benefits are well documented, some organizations remain stubbornly on the sidelines, concerned that the cost of launching and running a data analytics project will outweigh any potential rewards. Yet as data analytics technology grows ever more powerful and accessible, such enterprises may now want to reconsider their decision.
First steps
To introduce data analytics effectively, enterprises need to develop a strategy that promotes both top-down and bottom-up initiatives, said Gonzalo Zarza, director of data and analytics for IT and software development company Globant. "On one hand, top-down or management-driven initiatives play a major role in adoption ... through leading by example," he explained. "On the other hand, a sharp selection of bottom-up projects to be executed first has proven to help break initial reluctance and skepticism arising from different teams and well-established areas in the organization."
Begin the journey into data analytics by building a strong foundation, advised Rosaria Silipo, principal data scientist at KNIME, an open source data analytics company. "Hire key experts and choose the best software," she suggested.
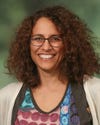
Rosaria Silipo, KNIME
Follow up by building an inventory of existing resources and capabilities, including whatever is available in the current data warehouse, the organizational structure and from staff competence. "A useful guide for this purpose is the Analytics Maturity Model developed by INFORMS, a leading academic and professional analytics organization," said Willem van Hoeve, a professor of operations research and head of the master of science in business analytics program at Carnegie Mellon University's Tepper School of Business.
The experts heading the enterprise's data analytics project should be versatile individuals with competences spanning the entire spectrum of data-related fields, including data analytics, data processing, data collection, data warehouses, data blending, data visualization, and data preparation. "It is, of course, hard to find one employee with expertise in all those disciplines," Silipo observed. "The effort should be distributed in order to hire a group of people to cover the global expertise."
Putting data to work
Most enterprises have collected a significant amount of data but don't really know it, since it's most likely siloed between different departments. "If they haven't done anything with data analytics, there's a good chance that individual departments have taken the initiative to build or purchase their own solutions," said Zach Reece, a former Deloitte CPA. Such an environment creates boundaries that need to be demolished.
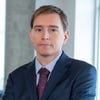
Willem van Hoeve, Carnegie Mellon University
Separating the analytics development process into stages can help new adopters to accurately assess costs, requirements, and the potential value of developing a data-driven analytical solution. "It also helps to suitably scope a project into milestones with measurable outcomes," Van Hoeve noted.
Choose a specific business problem that data analytics can solve, and build a solution for that problem, advised David Linthicum, chief cloud strategy officer for Deloitte. "An example would be inventory depletion and replenishment to avoid excess inventory costs," he said. "There are hundreds or thousands of these types of problems that enterprises can identify today."
Shervin Khodabandeh, a data analytics expert at management consulting firm Boston Consulting Group, recommended focusing on a handful of large initiatives, rather than several smaller projects, and securing senior management sponsorship. He dismisses the need to conduct multiple proof of concept projects. "Many proof of concepts fail not because the algorithms fail but because the initiative is not scoped, funded, staffed, and governed appropriately," he explained. "They get stuck in experimentation mode rather than value capture mode."
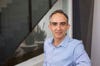
Shervin Khodabandeh, Boston Consulting Group
Avoiding mistakes
The most common mistake made by data analytics newcomers is focusing on the data instead of on the business problem at hand. Consider, for example, a logistics company that has equipped its truck fleet with GPS technology. Such a system will generate data showing the precise movements of each vehicle. That's all well and good, yet what the business really needs is data analysis that will help it optimize its delivery routes. "For that problem, we need entirely different data -- for example, demand data and inventory levels over time," Van Hoeve noted.
Another trap is falling for vendor marketing pitches. "The hype usually pushes you toward new, very promising, but still experimental technology, which will require quite an investment," Silipo said. "You need to evaluate whether the current hype is indeed what you need, or if the same goal can be achieved with more traditional, stable and less expensive data analytics techniques."
For more on data analytics, follow up with these articles:
Why Everyone's Data and Analytics Strategy Just Blew Up
10 Data and Analytics Trends for 2020
IT Disappoints Business on Data and Analytics
About the Author
You May Also Like