The Need for Inclusion in AI and Machine LearningThe Need for Inclusion in AI and Machine Learning
If companies are forward-thinking in their application of predictive analytics, AI, and machine learning they can make these technologies inclusive, and available and relevant to all people.
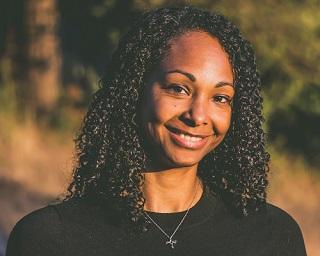
Imagine if something not designed with you or anyone like you in mind was the driving force of how regular interactions permeate your life. Imagine it controls what products are marketed to you, how you can use certain consumer products (or not), influences your interactions with law enforcement, and even determines your health care diagnoses and medical decisions.
There are problems brewing at the core of artificial intelligence and machine learning (ML). AI algorithms are essentially opinions embedded in code. AI can create, formalize, or exacerbate biases by not including diverse perspectives during ideation, testing, and implementation. Ageism, ableism, sexism, and racism are being built into some services that are the foundation of many “intelligent” systems that shape how we are categorized, advertised to, and serviced, or disregarded.
ML output is only as good as the repetitive data, pictures, and word association inputs. If those inputs are chosen by a small, homogenous group of engineers or product managers, with no refinement or review outside of that group, the output can create biased results with little ability to check the underlying logic. This implies that the selection of training data needs to give a complex (instead of a singular) view of history, which will inform the future. If the sample size for these technologies is small and uniform, algorithms will adopt and reinforce biases gleaned from incomplete data trends, pictures, and words.
We can see recent examples of this when homogeneous teams, testing, and data create problems. It appears in simple photo recognition systems, such as Google’s mishap during summer 2015, when its Photo app tagged two pictures of Black people as gorillas.
There are also examples of AI and ML being developed on top of data-driven insights, that if not inclusive could actually have damaging, and possibly life threatening, results. Earlier this year, the results of a University of Adelaide (Australia) study of 48 participants, all of whom were at least 60 years old, yielded 69 percent accuracy when predicting who would die within five years from the analyzed photos of people’s organs using artificial intelligence. While the ability to predict with such accuracy is phenomenal, there is a lesson to be learned when analyzing the small group of participants.
One can see how this information could be used for ML purposes to inform healthcare decisions and provide counsel and care. But key questions linger. What were the ethnicities of the participants? This is vital information, as certain ethnicities have greater cases of certain diseases and ailments, like black women have a higher instance of fibroids, and Latinx populations are more likely to have diabetes and liver disease. If the data sets that inform the technology do not include this kind of information, this could lead to disparate outcomes in health care treatment, including misdiagnoses, no diagnoses, or poor treatment plans.
Another sector where AI and ML can inform is public safety and police enforcement. Predictive policing applications, like Hunchlab, use historical crime data, moon phases, location, census data, and even professional sports team schedules to predict when and where crime will occur. The problem with that is the foundational element of historical crime data can be based upon policing practices that disproportionately target, for example, young black and brown men, primarily in low income areas. These practices have been confirmed via the Justice Department findings in Ferguson, Missouri, and recent task force findings in San Francisco. If historical data shows most crime and arrests are in poor minority areas, predictive technology will reinforce that data, thus perpetuating a cycle of over-policing in poor minority areas.
Applications imbued with intelligence can also determine how, when, and why you spend your money. Advertising agencies are testing IBM Watson’s AI capabilities to target offers for their clients' products. For example, a campaign personalizes recommendations by understanding a user’s personality, tone, and emotion conveyed in a conversation – all of which sounds great if the recommendations don’t blur into profiling. A hypothetical outcome to avoid: The application decides based on your vocal patterns that you’re more South Central Los Angeles than Beverly Hills and provides only information on historically black colleges and universities.
Let’s avoid this future and instead create an inclusive one. Here are practical steps to help.
Tap into a diverse team. If you don’t have representation from the different types of customers you serve (or want to serve) on the core team, can you find it on the extended team? Bringing in a diverse set of employees from other functions gives additional perspective. If you still can’t find a representative sample inside your company, how about bringing customers into the development cycle. You can get their feedback during user story creation or have them sit in on acceptance demos.
GoDaddy learned that lesson in building a recent product, a do-it-yourself website builder called GoCentral. It includes thousands of images that are queried to select the relevant few to build a first version of a small business’ website. When we launched the service in the US, almost all the images showed Caucasians when they featured people. Our customer care team and an early customer immediately pointed out that this did not work for websites selling to minorities. In addition, we had global aspirations for the product, so showing solely Caucasian faces, where other skin types are in the majority, wasn’t representative. The team threw out those images and started again, pulling in a much more diverse set of images.
Find a diverse training data set. If your objectives are to serve a broad audience, it is critical to expand your sources to get enough data for each segment. As voice-command user interfaces are growing in usage, there are many people who are frustrated because they are not understood. Why? Because they have an accent. Much of the early training data for voice services came from researchers who had collected data using neutral accents (like those of broadcasters) and college students (a homogenous group). Google’s voice recognition services are expanding the training set data, setting an example of how to tackle problems of narrow data sources leading to biased and therefore unusable services.
We’ve also found at GoDaddy that we need to explicitly obtain data that covers customers with enough depth to get good results for each group. An example is that our domain search modeling team initially applied our US model in deciding what domain names to show in other countries and saw it perform worse than having no “smarts” at all. Why? The international customers behaved quite differently when exposed to the same patterns. Only once we broke out specific countries into separate models did we see significant improvement.
The pace of law lags that of technology, as the latter drives innovation and the former waits to see the results before passing legislation or creating policies. If companies are forward-thinking in their application of predictive analytics, AI, and machine learning they can make these technologies inclusive without the need for new laws or regulations. There are clear steps available to all companies, regardless of size, to bring this technology to all people. It starts with someone on the team asking the question, “Are we building a vision of the future that includes everyone?”
Steven Aldrich is chief product officer at GoDaddy. It’s Steven's job to deliver innovative, integrated and differentiated products. Steven joined GoDaddy in 2012, initially leading GoDaddy’s Productivity business. He spent over a decade at Intuit, where he helped grow the consumer and small business divisions by moving them from the desktop to the web. He also co-founded an online service that simplified shopping for insurance and has been the CEO of two other venture-funded start-ups. Steven earned an M.B.A. from Stanford and a B.A. in physics from University of North Carolina. Steven serves as a member of the Board of Directors of Blucora.
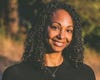
Bärí A. Williams, Esq. is Associate General Counsel at Marqeta. Previously she was Head of Business Operations Management, North America at StubHub, where she was responsible for business planning and operations to manage and oversee technical metrics, product innovation, and partnerships and drive P&L results across the company. Prior to StubHub, Bärí was a commercial attorney at Facebook supporting internet.org connectivity efforts, building aircraft, satellites, and lasers, along with purchasing and procurement.
About the Author
You May Also Like