Top Data Challenges Created by Digital TransformationTop Data Challenges Created by Digital Transformation
As data becomes more valuable to organizations, its usefulness for analytics means that more data needs to be stored for longer periods of time.
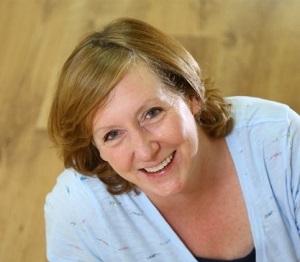
Data clearly is not what it used to be. Organizations of all types are finding new uses for data as part of their digital transformations. Examples of data becoming the key to competitive advantage abound in every industry, from jet engines and autonomous cars, to agriculture and grocery stores.
Not only are uses of data changing, but the types of data that enable new insights are also changing. This “new data” is very different from the financial and ERP data that we are most familiar with. That old data was mostly transactional, and privately captured from internal sources, and continues to be critical.
New data, on the other hand, can be transactional or unstructured, publicly available or privately collected, and its value is derived from the ability to store, aggregate and analyze all of it, quickly.
Although the opportunities to leverage new data are endless, organizations will face many challenges as they try to learn how to gain valuable insights and competitive advantages from it. CIOs and datacenter architects should consider the impact of new data on their datacenters and their investment strategy, because of its different characteristics and uses:
Data capture is widely dispersed. New data is captured at the source, and that source might be beneath the ocean in the case of oil and gas exploration; within satellites in the case of weather applications; within phones in the case of pictures and tweets; or in vehicles in the case of connected cars. The volume of data collected at the source will be several orders of magnitude higher than we are familiar with today. The example of a connected car is a good one, as each connected car is expected to generate up to 1 terabyte of data per day by 2020. Scale that for millions, or even billions of cars, and then we have an onslaught of new data.
It is clear that we cannot capture all of that data at the source and then try to transmit it over today’s networks to a central location for processing and storage. This challenge is driving the development of completely new datacenters, and a new “edge computing” environment that can capture, store and partially analyze large amounts of data locally prior to transmitting and aggregating it somewhere else.
New edge computing environments are going to drive fundamental changes in all aspects of the computing infrastructure -- from CPUs to GPUs and even MPUs (mini-processing units); to low power small scale flash storage; and to Internet of Things (IoT) networks and protocols that don’t require what will become precious IP addressing.
Data scale is exponential. The scale of large cloud providers is already such that they must invest heavily in automation and intelligence for managing their infrastructure. Any manual management is simply cost prohibitive at the scales in which they operate. This problem will become pervasive to all datacenters as the volume of data being stored and analyzed grows. Try to imagine using protocols, such as IPMI, to interrogate hundreds of thousands of devices as the way to identify failures! The likelihood that datacenter infrastructure will become very specialized to a single function will only exacerbate the management challenges.
Intelligent storage and infrastructure automation is the only answer. The same new technologies that will apply to data (such as predictive analysis and AI), will need to be applied to managing and maintaining the infrastructure as well. Expect to see a completely new type and heightened level of importance applied to infrastructure management in the future.
Data mobility is challenging network bandwidths. If data is everywhere, then it must be moved in order to be aggregated and analyzed. Just when we thought (hoped) that networks were getting faster than Internet requirements at 40 to 100 Gbps, data movement is likely to increase by a factor of 100X to 1,000X. In addition, the current cloud paradigm of making it free to store data, but cost prohibitive to take it out, is completely counter-intuitive to the new data requirements.
Data is the driver of the new economy, and will be very long lived. Processing against it in the form of analytics will be iterative and continuous. In the world of new data, we need to re-think the definition of network. For example, the work being done to create urban high-speed WiFi networks at large social media and Internet giants, will certainly be required in the future.
Data value is revolutionizing storage. There is no question that data is becoming more valuable to organizations and its usefulness over longer periods of time is growing as a result of machine learning and AI-based analytics. This means that more data needs to be stored for longer periods of time and that data must be addressable in aggregate in order for analytics to be effective. As a result, this is driving many new ways to store and access data, from in-memory databases to 100PB-plus scale object stores. We can expect these new architectures to replace the traditional storage paradigms of block and file storage, and SQL databases, over the next five years.
Analytics on new data will drive changes to computing infrastructure
If new data is more valuable through analysis, then analytics will be the driver for compute-intensive applications of the future. Analytics are available in many forms, from batch statistical analytics, to real-time streaming machine learning-based algorithms, and everything in between. Analytical applications are both I/O and compute-intensive. Since most analytics of this type are performed on unstructured data, they don’t use floating point instructions, thus reducing the need for such operations in the CPU chips.
Similar to other high-performance computing applications, analytics takes advantage of parallelism, making the use of GPUs an interesting option. Some analytics will happen at the edge, in order to reduce the cost of transmitting large amounts of data. For an edge-compute infrastructure, very low cost, special-purpose processing will be a critical component.
It is inevitable that these challenges will drive the evolution of datacenter architectures for the next five years. If digital transformation creates new data,then we must accept that new data creates a need for a new type of datacenter -- one that drives new measures for data value. These new datacenter architectures will be large scale, data centric, and employ a purpose-built infrastructure for compute, storage and networking.
Joan Wrabetz is the Vice President of Strategic Marketing for the Datacenter Systems business unit at Western Digital Corp. and leads the global marketing efforts for HGST-branded datacenter infrastructure products covering public and private clouds. Prior to joining Western Digital, Joan held senior executive positions at QualiSystems, EMC, Aumni Data (founder and CEO), Tricord Systems, StorageTek, and Aggregate Computing. She earned a Master’s of Business Administration degree from the University of California at Berkeley, a Master’s of Science degree in Electrical Engineering from Stanford University, and a Bachelor’s of Science degree in Electrical Engineering from Yale University. Joan also holds patents in load balancing, distributed systems, and machine learning classifications and analytics.
About the Author
You May Also Like