Unmasking the Black Box Problem of Machine LearningUnmasking the Black Box Problem of Machine Learning
Standard Chartered taps Truera to pull back the veil for better transparency on how its data gets analyzed and the predictions algorithms make.
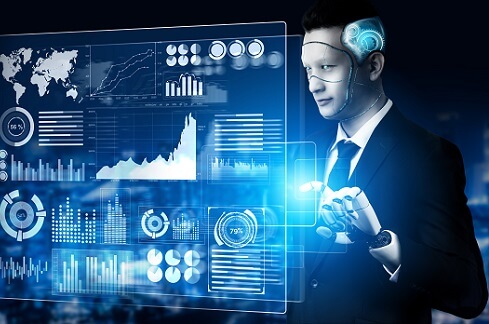
Financial and banking services company Standard Chartered turned to a model intelligence platform to get a clearer picture of how its algorithms make decisions on customer data. How machine learning comes to conclusions and produces results can be a bit mysterious, even to the teams that develop the algorithms that drive them -- the so-called black box problem. Standard Chartered chose Truera to help it lift away some of the obscurity and potential biases that might affect results from its ML models.
“Data scientists don’t directly build the models,” says Will Uppington, CEO and co-founder of Truera. “The machine learning algorithm is the direct builder of the model.” Data scientists may serve as architects, defining parameters for the algorithm but the black box nature of machine learning can present a barrier to fulfilling an organization’s needs. Uppington says Standard Chartered had been working on machine learning on its own in other parts of the bank and wanted to apply it to core of the business for such tasks as decisioning on when to offer customers loans, credit cards, or other financing.
The black box issue compelled the bank to seek greater transparency in the process, says Sam Kumar, global head of analytics and data management for retail banking with Standard Chartered. He says when his organization looked into the capabilities that emerged from AI and machine, Standard Chartered wanted to improve decision making with such tools.
Standard Chartered wanted to use these resources to better predict clients’ needs for products and services, Kumar says, and in the last five years began implementing ML models that determine what products are targeted for which clients. Wanting to comply with newer regulatory demands and halt potential bias in how the models affect customers, Standard Chartered sought another perspective on such processes. “Over the last 12 months, we started to take steps to improve the quality of credit decisioning,” he says.
That evaluation brought up the necessity for fairness, ethics, and accountability in such processes, Kumar says. Standard Chartered had built algorithms around credit decisioning, he says, but ran into one of the inherent challenges with machine learning. “There is a slight element of opacity to them versus traditional analytical platforms,” says Kumar.
Selection process
Standard Chartered considered a handful of companies that could help address such concerns while also maintaining regulatory compliance, he says. Truera, a model intelligence platform for analyzing machine learning, looked like the right match from cultural and technical perspectives. “We didn’t want to change our underlying platform for a new one,” Kumar says. “We wanted a company that had technical capabilities that fit in conjunction with our main machine learning platform.” Standard Chartered also wanted a resource that allowed for insights from data to be evaluated in a separate environment that offers transparency.
Kumar says Standard Chartered works with its own data about its clients, data gathered from external sources such as credit bureaus, and from third-party premium data resellers [with client consent]. How substantial particular pieces of data can be in driving an outcome becomes more opaque when looking at all that data, he says. “You get great results, but sometimes you need to be sure you know why.”
By deconstructing its credit decisioning model and localizing the impact of some 140 pieces of data used for predictions, Kumar says Standard Chartered found through Truera that 20 to 30 pieces of data could be removed completely from the model without material effect. It would, however, reduce some potential systemic biases. “You don’t always have the same set of data about every single client or applicant,” he says.
Relying on a one-size-fits-all approach to decisioning can lead to formulas with gaps in data that result in inaccurate outcomes, according to Kumar. For example, a 22-year-old person who had credit cards under their parents’ names and might not have certain data tied to their own name when applying for credit for the first time. Transparency in decisioning can help identify bias and what drives the materiality of a prediction, he says.
Black box problem
There are several areas where the black box nature of machine learning poses a problem for adoption of such a resource in financial services, says Anupam Datta, co-founder and chief scientist of Truera. There is a need for explanations, identification of unfair bias or discrimination, and stability of models over time to better cement the technology’s place in this sector. “If a machine learning model decides to deny someone credit, there is a requirement to explain they were denied credit relative to a set of people who may have been approved,” he says.
This kind of requirement can be found under regulations in the United States and other countries, as well as internal standards that financial institutions aspire to adhere to, Datta says. Professionals in financial services may be able to answer such questions for traditional, linear models used to make decisions about credit, he says.
Nuanced explanations can be needed for such results to maintain compliance when applying complex machine learning models in credit decisioning. Datta says platforms such as Truera can bring additional visibility to these processes within machine learning models. “There is a broader set of questions around evaluation of model quality and the risk associated with adoption of machine learning in high stakes use cases,” he says.
For more content on machine learning, follow up with these stories:
How Machine Learning is Influencing Diversity & Inclusion
About the Author
You May Also Like