When Good Algorithms, Tech Stop Being GoodWhen Good Algorithms, Tech Stop Being Good
What happens when an algorithm designed for good gets used for illegal purposes? Technology designed TO fix a problem can ultimately lead to negative consequences.
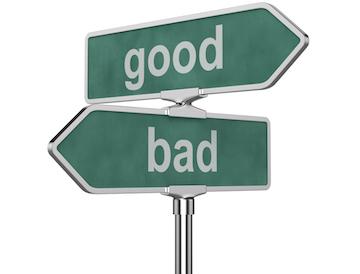
Back in August, All Analytics Editor in Chief Jim Connolly posted a blog that addressed the unpredicted use of Marc Elliott's algorithm, which was originally designed for medical research, but was also ultimately used to guess the race of people applying for credit (unbeknownst to the algorithm's creator). Jim's blog drew my attention because it is a great example of the potential onset of "technological iatrogenesis." Iatrogenesis is a medical term derived from Greek and it means "brought forth by the healer". Medically, it refers to the negative effects caused by medical or surgical procedures intended to help. In short, iatrogenesis refers to a cure that is worse than the disease.
In linking this concept to information technology in general, and for our purposes analytics in particular, technological iatrogenesis (TI) is when the deployment of a technical product, good, or service creates more problems than it eliminates. In that light, Jim's article caught my eye because it surfaced the risk that data scientists, analysts, statisticians, etc. could misapply algorithms and other tools under the assumption that one size fits all. Elliott designed his algorithm for one industry and to his surprise, it was being applied beyond the intended scope.
There are at least four ways that TI can occur. The first way assumes that a technology is impervious to time. Tape backups are a traditional way of storing mass quantities of data. And at one point, tape backups were a leading edge solution. But without migrating from tape (which degrades of time) at some point to digital or cloud service storage, historical business assets will be lost. Magnetic tape cured the storage problem in the past, but it inherently becomes a risk over time.
The second way that TI can occur assumes that embedded business rules are applicable across functional units, industries, or broad categories of analysis. For example, if the business rules of your system seasonally adjusts changes in employment based on the industry (retail store hiring may change when summer school students are available for the labor market), those same rules may produce deleterious results if you use them for seasonally adjusting changes based on geography. Rules based on what an establishment does are not suitable for adjustments based on where the establishment is located.
The third way that TI can occur assumes that data definitions have transitive properties and are vehicular in nature; this is the assumption that all terms are synonymous and are transferable across all systems. For example, a database variable labeled "hotness," which was designed for a dating service system would have a whole different meaning if used for a system designed to predict menopause symptoms. In like manner, a database variable labeled "nova," would have an astronomical meaning to a space scientist, a marketing meaning to a Chevrolet sales manager and quality meaning to automobile customers in Latin America.
The fourth way that TI can occur assumes that an IT solution designed for decentralized processing can be easily migrated into a centralized processing architecture. Decentralized systems are typically self-contained and have direct access to whatever processing resources are needed, whenever the resources are needed, for as long as the resources are needed. Centralized systems typically share some resources, even if configured as a series of virtual machines. Granted, centralized systems may be cheaper, but they work best when all of the processing nodes are intentionally designed in that manner from the start. Attempting to indiscriminately integrate a decentralized solution into a centralized architecture is not wise and will be more expensive than designing a "plug and play" architecture from the beginning.
In these days when organizations are seeking sharable and reusable solutions, it makes sense to avoid reinventing the wheel. But great care, reflection and investigation needs to occur to prevent the desired cures from being worse than the illness. While analytic professionals do not take the Hippocratic Oath, we should in some fashion observe this saying: Primum non nocere -- "First, do not harm".
Read more about:
2017About the Author
You May Also Like