Where Common Machine Learning Myths Come FromWhere Common Machine Learning Myths Come From
There are a lot of misconceptions about ML that can have a negative impact on one's career and reputation. Forrester and ABI Research weigh in.
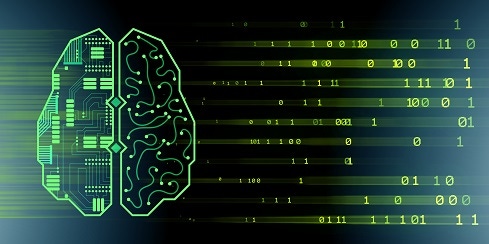
Forrester Research recently released a report entitled, Shatter the Seven Myths of Machine Learning. In it, the authors warn, "Unfortunately, there is a pandemic of ML misconceptions and literacy among business leaders who must make critical decisions about ML projects."
When executives and managers talk about AI and machine learning, they sometimes make factual mistakes that reveal their true level of knowledge. Forrester senior analyst Kjell Carlsson, who is the lead author of the report, said in a recent interview that he's heard audible sighs over the phone when experts hear what lay people have to say.
"When the head of product says something like, 'We're using reinforcement learning because we're incorporating user feedback into the trends modeling,' that's probably not a good thing," said Carlsson. "I've been on panels with other analysts and I'm hearing thing like, 'With unsupervised learning you no longer need humans involved or training' and you're like, wait, what?"
ABI Principal Analyst Lian Jye Su said in his experience, most executives have some sort of ideas around the basics of machine learning and the "garbage in, garbage out" principle, but most of them believe machine learning models are black boxes and that machine learning requires massive amounts of data.
"I would argue that this is mainly due to the prevalence of convolutional neural networks that require large amounts of data and somehow work better with extra numbers of convolutional layers, and I believe such perceptions will slowly disappear once other machine learning algorithms become more popular," said Su.
One issue is education. Exactly where should decision makers learn the truth about machine learning? There are lots of practitioner and business-level options, although the intersection of the two is what Forrester's Carlsson thinks is missing.
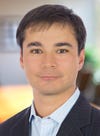
Kjell Carlsson, Forrester
"Where I think we need the most work and the most help is helping folks from the business side understand the technology enough to know what is this actually good for? What sort of problems can I apply it to?" said Carlsson.
Following are some of the factors that lead to common misperceptions.
The terminology is not well-understood
Part of the problem is the terminology itself. People sometimes interpret artificial intelligence as machines that think like people and machine learning as machines that learn like people.
"Data scientists are not the best at nomenclature," said ABI Research's Su. "I would argue we analysts are partially to blame for this, as we often use big words to introduce new technologies."
Unrealistic expectations
There is a general misconception that AI is one, big powerful thing, which leads to the belief that AI can do anything. Alternatively, deep learning is sometimes interpreted as "better" than other forms of machine learning when different techniques are suited to different types of use cases.
It's not very helpful to just start with what you want like replacing everyone in the call center with a virtual agent," said Forrester's Carlsson. "They're much more set up in an augmenting fashion to help somebody in the call center."
ABI Research's Su said unrealistic expectations is one case in which hype takes over rational thinking. In his experience, executives are thinking less and less about expecting the impossible or the improbable.
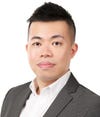
Lian Jye Su, ABI Research
Failure to understand the probabilistic nature of machine learning
Traditionally, software has been built deterministically, meaning a given input should result in a given output. The same is true for rules-based AI. On the other hand, machine learning has a margin of error.
"In the machine learning world, it's perfectly possible that you'll never be able to predict the thing you want to predict because the signal isn't in the data you have," said Forrester's Carlsson.
ABI Research's Su said one of the arguments against using machine learning is the probabilistic nature of the outcome. It's never as clear cut as the conventional rules-based AI used in industrial machine vision.
Overlooking important details
An engine manufacturer wanted to predict when parts needed to be replaced. The company had an abundance of data about engines and engine failures, but all of the data was lab data. There were no engine sensors operating in the field. So, the model couldn't actually be deployed as intended.
"There's really no one in the organization who oversees all of the different things on the data engineering side, the machine learning side," said Forrester's Carlsson.
There's also a bit of common sense that comes into play that can get lost between technological capabilities and the ROI of those capabilities. For example, models have been built that recommend good accounts for salespeople to call. The problem is that the salespeople were already aware of those accounts.
Failing to understand what machine learning ‘success’ means
Laypeople often expect more from machine learning and AI than is practical. While 100% accuracy may seem reasonable, considerable time and money can be spent eking out yet another 1% accuracy when the use case may not require it.
Context is important. For example, accuracy levels differ when someone's life or liberty is at stake versus the possibility that a percentage of a population might be mildly offended by something.
"There is an entire school of thought around quantization, where, depending on the nature of the AI tasks, a reasonable level of reduction in the accuracy of AI models can be acceptable as a trade-off, provided this allows AI to be deployed on edge devices," said ABI Research's Su. "After all, we humans are often not as accurate. Having said that, certain applications such as object classification, defect inspection, and quality assurance on the assembly line do have stringent requirements that demand repeatability, and this is where conventional rules-based AI is probably preferred."
Forrester's Carlsson said everyone can create a model that would pretty much result in 99.99% accuracy. Predicting terrorism is one example. It happens so infrequently that if the model predicted no terrorism all of the time, it would be a hyper-accurate model.
Failing to go after easy wins
Science fiction and advertisements lead people to believe that they should be doing something remarkable with AI and machine learning when there's a lot of value to be had in use cases that aren't very sexy.
"When you say machine learning or AI people automatically think that they should be going to something which is mimicking human behavior and that's often missing the vast potential of the technology," said Carlsson. "Machine learning technologies are really good at working with data at scale and doing analysis at scale that we humans are really terrible at."
7 tips to keep in mind
1. Understand the capabilities and limitations of machine learning, and to some extent the uses cases to which different techniques are suited. That way, you're less likely to say something that's technically inaccurate.
2. One machine learning technique doesn't fit all use cases. Classification use cases, such as identifying pictures of cats and dogs differ from finding a previously undiscovered signal in data.
3. Machine learning is not a collection of "set and forget" techniques. Models in production tend to "drift" which means they become less accurate. Machine learning models have to be tuned and retrained to even maintain their accuracy.
"In software development, there's this understanding about the need to be iterative," said Forrester's Carlsson. "When it comes to applications which are relying on machine learning models, they have to be even more iterative because you're iterating on the data, the business use case and the methods that you're using in tandem. None of them are ever really fixed at the beginning of a project because we don't know what data you have, or you don't know what business use cases that data could support."
4. Machine learning accuracy is relative to the use case. In addition to considering the risks associated with potential errors, realize the art of the possible changes over time.
"A 50.1% computer vision model is wonderful. Or you may say 60% accuracy or 70% accuracy is way better than anything we've done before," said Carlsson.
5. Context is crucial. AI and machine learning can't achieve the same results irrespective of context. Context determines the techniques that are better or worse and the level of confidence that's acceptable or unacceptable in a given situation.
Context also has a bearing on what data is required to solve a certain problem and whether biases are acceptable or unacceptable. For example, discrimination is considered a bad thing, generally speaking, but it's understandable why a bank wouldn't loan just anyone millions of dollars.
"In many cases, machine [learning] is definitely bad at identifying past biases that were hidden in data. In other cases, the quality of the data matters, such as pixel count, clear annotation, and a clean data set," said Su.
On the other hand, the cleanest data isn't helpful if it's the wrong data.
"Folks are assuming that machine learning and even AI is going to somehow do something magical when the data is not around and that that doesn't work. [Conversely,] folks are assuming that as long as we have lots and lots of data, we will be able to do something magical, which often doesn't hold either, said Forrester's Carlsson. "Having bad quality data on the right thing [can] actually [be] better than having massive amounts of data on the wrong thing."
6. Understand that machine learning is a combination of hardware and software. Specifically, ABI Research's Su said the software capabilities will only be as good as what the hardware can deliver or is designed to deliver.
7. Conventional rules-based AI will likely co-exist with machine learning-based AI for quite some time. Su said some tasks will continue to require deterministic decision-making instead of a probabilistic approach.
For more about machine learning in the enterprise check out these articles.
How to Manage the Human-Machine Workforce
About the Author
You May Also Like