Why Operationalizing Analytics is So DifficultWhy Operationalizing Analytics is So Difficult
Plenty of companies have plenty of data and plenty of analytics tools, but they fall short when it comes to converting analytics results into action.
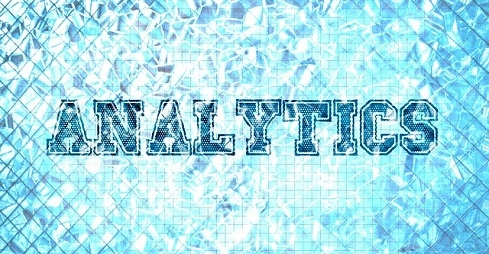
Today's businesses are applying analytics to a growing number of use cases, but analytics for analytics' sake has little, if any, value. The most analytically astute companies have operationalized analytics, but many of them, particularly the non-digital natives, have faced several challenges along the way getting the people, processes and technology aligned in a way that drives value for the business.
Here are some of the hurdles that an analytics initiative might encounter.
Analytics is considered a technology problem
Some organizations consider analytics a technology problem, and then they wonder why the ROI of their efforts is so poor. While having the right technology in place matters, successful initiatives require more.
"The first key challenge is designing how and in what way an analytics solution would affect the outcome of the business," said Bill Waid, general manager of Decision Management at FICO. "We start by modeling the business problem and then filling in the analytic pieces that address that business problem. More often than not, there's a business process or business decision that needs to be incorporated into the model as we build the solution."
Framing the business problem is essential, because if the analytics don't provide any business value, they won't get used.
"Better than 80% of analytics never end up being used. A lot of that stems from the fact that an analysis gets built and it might make sense given the dataset but it's not used to make something happen," said Waid. "That's probably the hardest element."
Placing analytics in the hands of the business requires access to the right data, but governance must also be in place.
"[T]he technical aspects are becoming easier to solve and there are many more options for solving them, so the people and the process challenges that you'll face obviously have to come along," said Bill Franks, chief analytics officer at the International Institute for Analytics (IIA). "In a non-digital-native company, the people and process progress does not match the technology progress."
Operationalizing analytics lacks buy in
Many analytics initiatives have struggled to get the executive and organizational support they need to be successful. Operationalizing analytics requires the same thing.
"When you operationalize analytics, you're automating a lot of decisions, so the buy-in you require from all of the various stakeholders has to be high," said IIA's Franks. "If you're a digital native, this is what you do for a living so people are used to it. When you're a large, legacy company dipping your toe into this, the first couple of attempts will be painful."
For example, if an organization is automating what used to be batch processes, there need to be more safety checks, data checks, and accuracy checks. Chances are high that everything won't be done right the first time, so people have to get comfortable with the concept of iteration, which is just part of the learning process.
Analytical results are not transparent
If your company operates in a regulated environment, you need to be able to explain an analytical result. Even if you're not in a regulated industry, business leaders, investors and potential M&A partners may ask for an explanation.
"We refer to it as 'reasoning code' or 'the outcomes,' but in AI it's a form of explainable AI where you can explain to a business owner or a business user why the analytics came to the conclusion it came to," said FICO's Waid. "The second thing that you need to provide the business person with is some kind of dashboard for them to be able to change, adjust or accommodate different directions."
About the Author
You May Also Like