Why Your AI Pilot Fails to LaunchWhy Your AI Pilot Fails to Launch
Chances are your AI pilot project won't make it to production deployment. How come?
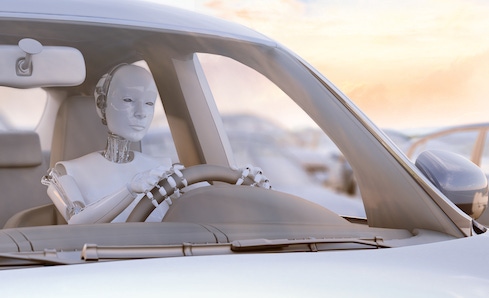
AI is important to your company's strategy going forward, and the executive team has earmarked increased investment in artificial intelligence and related technologies such as machine learning or natural language processing. There are plenty of surveys that show AI is part of the big strategic plan for enterprise organizations like yours. Yet many organizations appear to be stuck right when they get started.
While 9 out of 10 executives say that AI will be essential to their business strategy going forward, many executives are still trying to figure out how to fit AI into their business models and strategies.
Many organizations are getting stuck when it comes to their first forays into AI today. Pilots and initiatives aren't often turning into production deployments. There are a few reasons why these pilots fail to launch, according to analytics and AI icon Tom Davenport. Davenport is a professor at Babson College, a fellow at the MIT Initiative on the Digital Economy, and a fellow at NewVantage Partners.
"There are all different types of AI," Davenport told information in an interview. The category includes machine learning, deep neural networks, and natural language processing. Some people also include robotic process automation in the AI category, Davenport said. Each of these have their own challenges and issues regarding large scale implementation.
But generally speaking, if you are implementing one of these systems, they will touch your existing systems, too, and impact them in new ways. It's not just adding a new element to the system. It means a bigger transformation as the entire system may be required to change to accommodate the new element.
For instance, Davenport noted that while it's relatively easy to develop machine learning models, the percentage that actually get implemented isn't that big.
"It's because that involves changing or embedding some new code or at least a link to an API in your existing systems. And probably it involves some changes in behavior on the parts of some users," Davenport said.
As for natural language processing, there are many examples of companies that are experimenting with the use of chatbots internally in departments like HR and IT, Davenport said, but relatively few that have been willing to unleash those chatbots into a customer-facing environment without some sort of backup plan that lets the customers still talk to an actual human instead.
So many of these projects continue to feel like they are tentative, non-committal steps towards implementing AI or related technologies on a wide scale. Davenport said there are two main factors contributing to the inertia.
"Partly it's the stage of technological maturity, and partly, any big change involves people changing what they do, whether it's customers or employees or whatever," he said. Change is never easy, and workers and customers often resist things that are new.
Whatever the reason, the move into AI is happening, albeit slowly. In a recent post for MIT Sloan Management Review, Davenport writes that change management is a big issue for these projects.
"Most AI systems still involve some interaction with human workers, and educating those workers on new tasks and new skills can be time-consuming and expensive," Davenport and his colleague Randy Bean, CEO of NewVantage Partners, write.
The post also lays out some recommendations for speeding up the progress of AI implementations to production environments. For instance, organizations should set a time and criteria in advance for deciding whether to go into production. They can focus on adopting technology that can scale and be used by an intended audience such as customers. And they can adopt AI capabilities that are already embedded inside the transaction tools already in place in their businesses, such as Salesforce, SAP, Oracle, and Workday.
All these recommendations can be aligned with Davenport's other advice about choosing between AI projects that are "moonshots" vs. "low hanging fruit."
"I have a pretty strong belief that the vast majority of projects should not be moonshots -- highly ambitious things that require a lot of technical effort and a lot of organizational change. Maybe we'll see more moonshots, but if so, I think we'll see a lot of failures."
To learn more about the move to artificial intelligence in the enterprise, check out these recent articles:
Enterprises Wade Into the AI Pool
About the Author
You May Also Like