CIOs Need AI Platforms, Not Just ToolsCIOs Need AI Platforms, Not Just Tools
Open source tools are crucial to machine learning development, but if you want to manage those models in the enterprise, you need a platform.
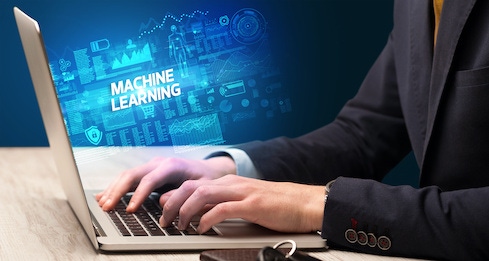
Trying to do more with less during the pandemic? While organizations may not be jumping into big investments right now, everyone is looking to conserve cash and maximize revenue in these uncertain times. Artificial intelligence and machine learning can be a part of achieving those goals, but there are some challenges to gaining the benefits.
"Machine learning relies on open source," Bradley Shimmin, Omdia analyst for data and analytics, told information. (Omdia and information are both owned by Informa) "In terms of turning that open source into an actual solution in the enterprise, it takes some doing."
A new report from Omdia can help provide a roadmap for organizations looking to gain those benefits quickly. The analyst research firm broke out some of the leading platforms to help organizations move early efforts to machine learning at scale with a platform approach.
The report names a handful of vendors from across the spectrum of platform providers as leaders in the space, to give organizations a sense of their options for managing machine learning at scale in the enterprise.
Shimmin noted that the vendors selected as leaders don't always compete with each other, and they may represent different specialties in the field.
But what all of these players will help organizations do is "turn what is a multi-year investment into something you can do in a shorter time. AI and ML can optimize business and drive new areas of innovation," Shimmin said.
"Given the fact that so many industries are trying to respond to a global pandemic makes that idea even more important," he said. "If your survival as a company depends on your ability to innovate quickly, find a new revenue stream, and extract every bit of value you can, AI and ML really can offer that."
The platform approach is a little different from where many machine learning professionals started. In school and at startups they built their project portfolios by using open source tools and libraries. But evolving any project from experimentation with a series of models to something that can be integrated with enterprise decision-making and operations takes a whole other level of effort.
Some pundits have argued that the wide array of open source tools, while brilliant for developing these individual projects, don't meet muster when it comes to coordinating and managing a machine learning practice for deployment at scale.
Organizations are coming to recognize that these open source tools and libraries hold an important place in a larger ecosystem of machine learning technology within enterprises. Yet the real power of these tools can only be felt when a full platform can be deployed to wrangle the tools and models. Open source and enterprise platforms must be used together.
"To create meaningful ML applications, it is necessary to understand the data that goes into an application, its provenance, how it is pre- and post-processed," wrote report author Michael Azoff. "...We talk of platforms rather than tools because these solutions span the whole ML development lifecycle and typically encompass multiple tools that are ideally accessed from one studio or console environment."
Omdia looked at a selection of eight companies across the spectrum of machine learning platforms. For public cloud companies it considered Microsoft and IBM. For a long-established analytics and ML vendor it looked at SAS. For relatively new ML vendors for general development it looked at C3.ai, Dataiku, H20.ai, and Petuum. And for a relatively new ML vendor dedicated to one task it looked at Evolution AI.
While the list is not exhaustive, Azoff notes, it "should provide a starting point for shortlisting vendors for further evaluation and proof-of-concept trials." All the platforms covered in the report provide support for the full ML lifecycle, according to Azoff.
That said, most of the companies included in the report were ranked as leaders, including Microsoft, SAS, IBM, C3.ai, and Dataiku. H20.ai and Petuum were challengers, and Evolution AI was a follower. Shimmin said that future reports will look at other technologies for machine learning, including Amazon SageMaker suite.
As for enterprise response to the pandemic, Shimmin said the anecdotal evidence he's seen so far is that investment in AI and machine learning has not slowed, and that it may be increasing.
"Those solutions can optimize your business to cut costs and make you more resilient to the change we are seeing now," he said. "It can also help drive new business which can also make you more resilient. It really can drive resiliency across highly disruptive market changes."
For more on AI and machine learning, check out these articles:
Using Analytics to Improve IT Operations and Services
Automating and Educating Business Processes with RPA, AI and ML
Adapting Cloud Security and Data Management Under Quarantine
About the Author
You May Also Like