Reducing the Environmental Impact of Artificial IntelligenceReducing the Environmental Impact of Artificial Intelligence
By adopting energy-efficient architectures, optimizing AI models for performance, and pushing for cloud providers to embrace renewable energy, businesses can help reduce the carbon footprint of their AI solutions.
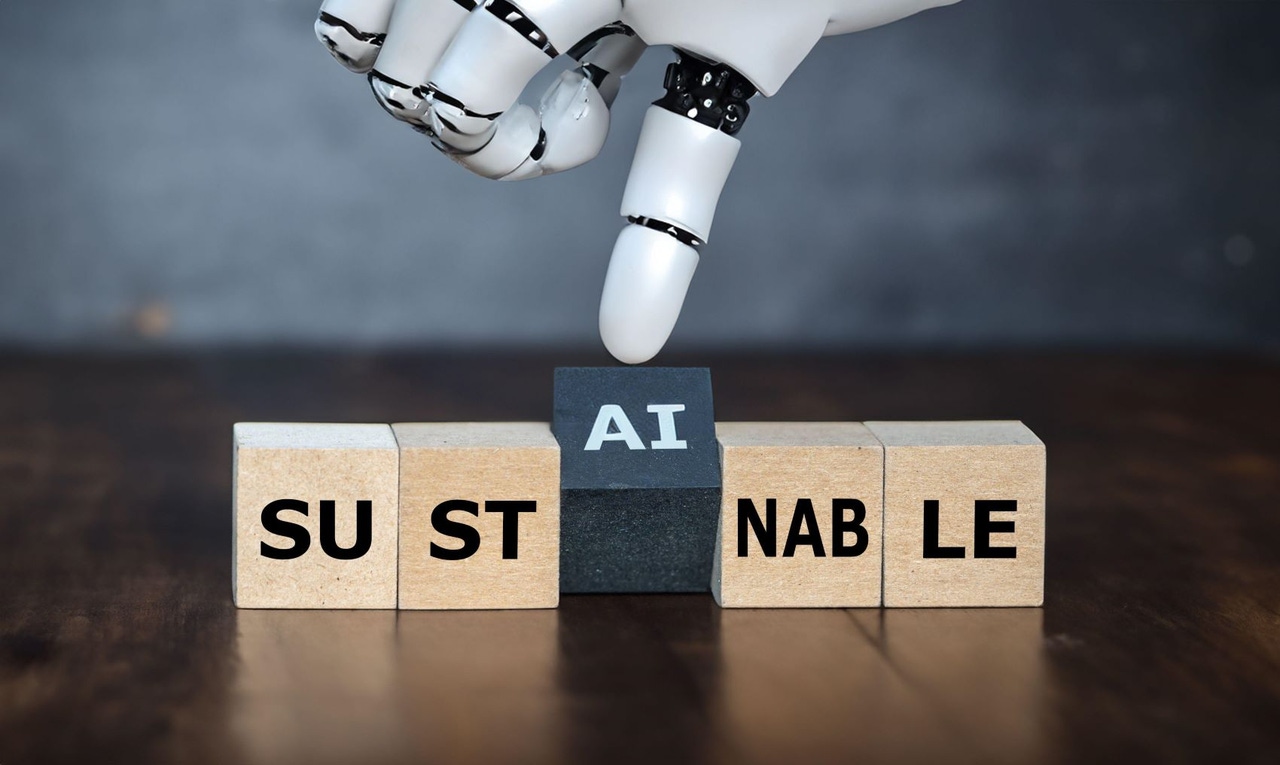
Artificial intelligence is reshaping our world. Its transformative power fuels innovation across industries -- delivering new value to organizations and consumers alike. As the proliferation of AI accelerates, people are starting to ask important questions: How does AI impact the environment? And furthermore, how do we keep pushing for progress without leaving a heavy carbon footprint on the planet?
AI’s Eco Impact
Artificial intelligence software runs in data centers that consume large amounts of energy and often cause significant carbon emissions. According to Bloomberg, there are more than 7,000 data centers worldwide. Collectively, they can consume as much power annually as the entire electricity production of Australia or Italy. The growing use of AI will further increase this already substantial energy consumption of data centers.
The use of AI can be separated into two main tasks: training and inferencing. During training, AI models learn from vast amounts of data that can take months depending on data complexity and volume. Once an AI model has been trained, it consumes energy each time it generates a new response or “inference.” The International Energy Agency (IEA) has reported a ChatGPT inquiry requires up to 10 times the electricity of a Google search to respond to a typical request. This energy consumption adds up and can quickly surpass the energy used for training.
The World Economic Forum estimates training comprises about 20% of an AI model’s overall energy use across its lifespan, while inferencing makes up the remaining 80%. AI’s overall environmental impact depends on model size, complexity, query volume and its energy source, although data on algorithm energy use remains limited.
Mindful Model Development
As organizations scale AI, understanding the factors influencing its environmental footprint can help address environmental challenges. Specifically, strategic planning in an AI's design phase can minimize the environmental impact across its lifespan. Considerations for organizations looking to develop energy-efficient AI models include:
A model’s platform architecture determines how efficiently it will use underlying hardware resources. This also influences the model’s overall resilience and long-term maintenance. Organizations decide where the processing will physically take place and what processors will do the work. Opting for energy-efficient architectures can help insulate businesses from rising AI-related energy costs, and growing future power demands for their solutions, which incur environmental costs even when relying on renewable energy.
Application design also impacts power requirements. Choosing a foundational model, instead of training a new one, avoids much of the energy needed for development and spreads the energy that is used across the model’s life. Techniques like quantization (compressing models to reduce memory usage of parameters) and dimensional reduction (transforming data from a high-dimensional space to a low-dimensional space) streamline processing and can further improve model efficiency. In some cases, AI applications can also be designed for batch processing instead of real-time processing, which tends to be more energy intensive.
Solution architects optimizing energy efficiency should aim to build the smallest and most efficient AI models necessary to achieve desired outcomes. Smaller language models perform faster and require less time and energy to process tasks. Building models of the “right-size” reduces energy requirements without sacrificing performance.
The training and retraining frequency of a model should also be considered. Companies can choose energy-saving model training methods like retrieval-augmented generation (RAG). RAG connects an AI neural network to a new knowledge base (such as a new technical paper or a database of images) without retraining.
Designing models for longevity can reduce their environmental impact by avoiding the need for retraining and redeployment. A generative AI model can produce millions or even billions of inferences over its lifespan. The number of processors supporting the model, along with their speed and power draw, influence the energy needed to produce each inference. A model seeing more traffic will generally require more energy than a less active one.
The Economics of Greener AI
AI is typically deployed in the cloud, where software-as-a-service (SaaS) providers rely on public cloud platforms to deliver AI-powered solutions. Different stakeholders in this ecosystem -- SaaS providers, cloud platforms and customers -- each have economic reasons to prioritize more environmentally friendly AI practices.
For SaaS companies, the cost of public cloud platform services and resources (like computing, storage and network capacity) directly affect margins. The more efficiently their AI models operate, the lower their resource consumption, which reduces costs and environmental impact.
Since AI models can be resource-intensive, minimizing usage through mindful model development becomes critical for both cost-effectiveness and sustainability. Public cloud platforms share similar incentives. Their profitability hinges on optimizing the procurement and operation of their data centers. Reducing energy consumption across computing and storage capacities leads to higher efficiency and better margins.
However, as AI usage grows, demand for public cloud resources will increase, leading to a significant rise in energy consumption -- even with optimized deployment. Therefore, the use of renewable energy to power public cloud platforms will be crucial to further reduce carbon emissions caused by AI and other cloud software.
This highlights the role of customers which have a growing influence over greener AI practices. With sustainability initiatives, regulatory pressures and consumer demands for transparency, many companies now prioritize vendors who demonstrate environmental responsibility. These organizations have the buying power to demand AI solutions that minimize energy consumption, pushing cloud providers toward greener operations such as running on renewable energy.
Ultimately, as more companies demand environmentally conscious AI, it will drive broader adoption of greener practices across the technology ecosystem.
The Path to Sustainable AI
By adopting energy-efficient architectures, optimizing AI models for performance and pushing for cloud providers to embrace renewable energy, businesses can help reduce the carbon footprint of their AI solutions. Sustainable AI isn't just about protecting the planet, it’s also a smart business move that can decrease costs and meet the rising demand for responsible technology from both regulators and consumers. The future of AI is bright but only if we ensure its green.
About the Author
You May Also Like