Unlocking the Full Value of One of Your Organization’s Greatest AssetsUnlocking the Full Value of One of Your Organization’s Greatest Assets
Organizations that invest in data strategy are most equipped to succeed in the age of AI. As technology capabilities grow, businesses must have a plan in place to not just use, but also optimize, their data.
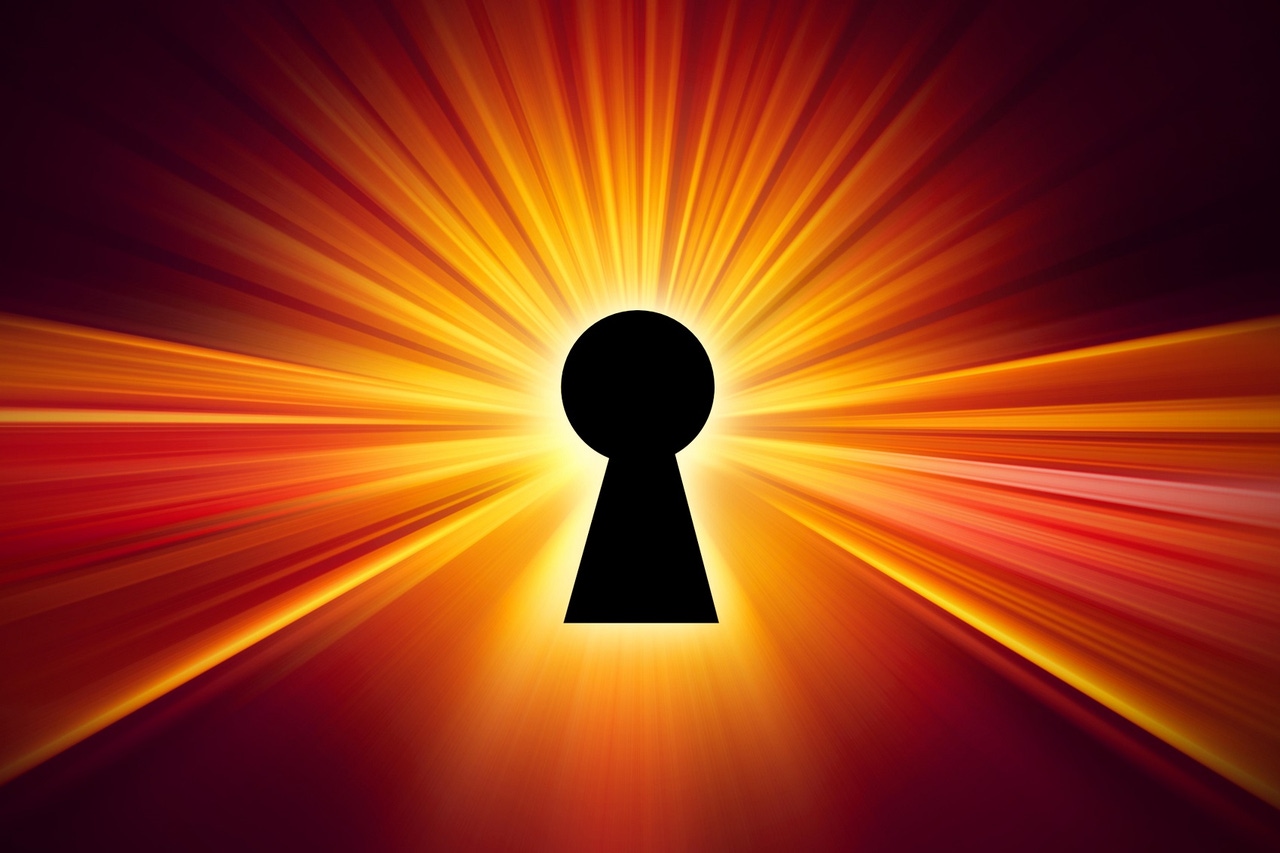
Land, equipment, inventory, cash. When a business organization considers its assets today, there is an important rising star to add to that list: data. Sometimes its value lies in buying and selling it, but its greatest worth is increasingly determined by its usefulness to internal operations. The International Federation of Accounting, for example, has suggested that data can be worth up to 30% of a company’s market capitalization.
Despite acknowledgment of its importance, data’s merit as a key intangible in planning and decision-making continues to be overlooked. A 2023 EY survey showed that 35% of C-suite respondents in large organizations had no data strategy and 45% were just getting started on developing one.
The introduction of early artificial intelligence tools over the past decade accelerated the emphasis on data gathering and use, but the capabilities and scope of generative AI (GenAI) over just the past two years has significantly amplified data’s value as a strategic resource -- fueling the growth and value engine.
However, if not given the planning and training focus it deserves, an underdeveloped or absent data strategy can pose serious threats, including lost revenue, brand and legal risks, and loss of stakeholder trust. Now is the time for decision-makers to focus on harnessing the full potential of their organizations’ data by having a data strategy that does the following: aligns with business goals; leverages data analytics tools to improve decision-making; uncovers new opportunities for innovation by identifying patterns and insights previously hidden; ensures management by the best resources; affirms quality inputs and outputs; and serves as a vital mechanism for meeting shifting legislative and regulatory requirements. Effective, AI-driven operating models for finance and tax functions, for example, simply cannot exist without a strong, data-driven foundation.
Developing an effective data strategy is a fundamental building block for achieving relevant and competitive tech transformation, and it requires investment, planning, and collaborative coordination among key C-suite stakeholders -- and benefactors, particularly CFOs and CIOs/CTOs.
Steps in Executing a Data Strategy
Executing a data strategy has turned into an art form in recent years. Consider the example of open banking, which is set to reshape financial services. Beyond expanding access to online payment options, it now offers secure sharing of all types of financial account data to other financial institutions, currency exchanges, merchants and digital platforms. The consumer is greatly empowered, even as the risk of shared access is potentially increased. So, too, the advancement of cloud technology platforms and system-to-system connectors by some of the biggest brand names in technology, means that data is now more accessible than ever. It is no longer trapped under the silo of any one system.
However, these advancements come with their own set of additional challenges as C-suite leaders look for detailed assurance and increased consistency in the usability of AI for decision-making. To overcome these challenges, we subscribe to five key stages in delivering an effective data strategy that makes the most effective use of AI:
1. Assess to identify
This first step involves gaining an understanding of how the current state of existing data elements are used for various processes and systems. The purpose of this step is to initiate a sufficiency analysis, often achieved via a data assessment. This determines whether there is a need for enrichment by identifying and bridging existing and potential future gaps.
Following the data assessment, identifying business requirements and performing a fit-gap study for data attributes as it pertains to concerned finance and tax business processes is necessary to convert the findings into an actionable plan.
2. Source to collect
Once an organization has the data roadmap, it should have the necessary ingredients to tackle the next step, which is sourcing of raw data from potentially disparate locations for the purpose of data collection. While developing blueprints, consider data storage, processing and analysis of data, as well as viability for cloud and on-premises hosting for data. During data collection, care needs to be taken to ensure that the data is at the right level of detail and quality for accuracy, completeness, consistency and timeliness. This ensures data is reliable and trustworthy prior to it being shaped for consumption.
3. Integrate to process
Classic extract, transform, and load (ETL) processes are performed in this stage to convert data to the desired format(s) and prepping for consumption. Data can be combined from one or more sources, and it can be distributed to one or more destinations.
4. Govern to manage
Data governance establishes clear policies and procedures for managing data throughout its lifecycle, ensuring data quality, security, privacy, and compliance with regulations. It sets the ground rules for risk management around its effective use, providing the rules for synchronization, reuse and shareability. Consider identifying data stewards who establish these metrics and track their performance.
5. Enable to action
The final stage involves making data actionable. This can vary from enabling the data for reporting/analytics, to enabling further automation and activation for complex use cases such as GenAI and ML. This is the stage where the data is “AI-grade” and ready for delivering business intelligence, leveraging analytical tools and techniques to extract insights, enabling informed decision-making and supporting strategic planning. At this juncture, business leaders can begin looking at AI applications that further drive value creation.
Continuous Improvement
While there is a logical purpose and benefit to following a methodology for enacting a data strategy, in fact, Step 5 should inform back to Step 1 to create a positive feedback loop. This feedback mechanism is critical to making certain that once a data strategy is enacted, it evolves and scales effectively to keep up with business, technological, and legislative demands.
Organizations that invest in data strategy and management of their information assets are well poised to achieve the agility needed to succeed with AI. Focusing on the key stages described above will help realize the value you seek and help internal teams work together to enhance and leverage the ongoing loop of quality data collection, management, and use.
The views reflected in this article are the views of the authors and do not necessarily reflect the views of Ernst & Young LLP or other members of the global EY organization.
About the Authors
You May Also Like