Is Synthetic Data the Future of AI Model Training?Is Synthetic Data the Future of AI Model Training?
As AI models require more and more information, synthetic data might be the answer. But users have both benefits and risks to consider.
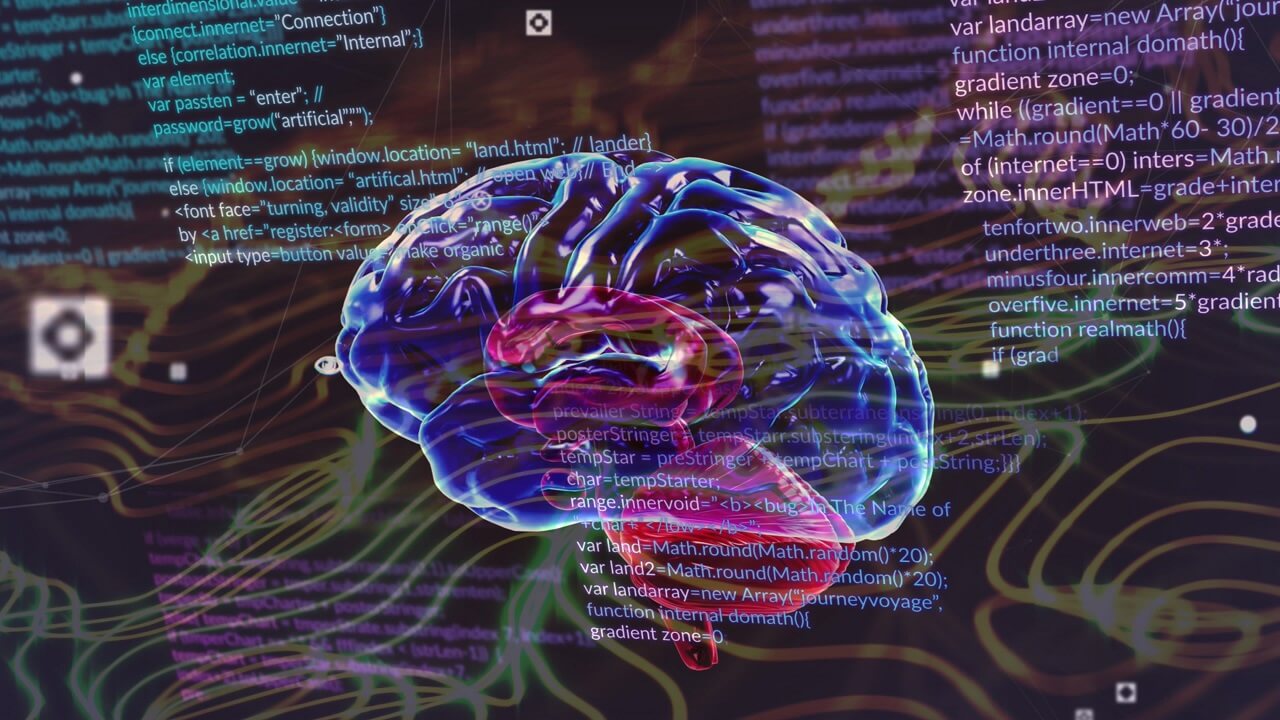
AI and machine learning are ravenous, and only data can feed their appetite. We produce more data than ever, but what is available for training large language models (LLMs) is not an unlimited resource. Research institute Epoch AI estimates that LLMs will run through public, human-generated data sometime between 2026 and 2032.
Given the money poured into the AI space, its stakeholders will hardly wait around to find out what happens if that estimate proves accurate. Epoch AI points to synthetic data as a possible innovation that could solve this problem.
“One way of describing it is it's data that you don't have but you wish you did,” Chris Hazard, PhD, cofounder and CTO of Howso, an understandable AI platform, tells information.
Real-world data is generated by people and actual occurrences, while synthetic data is generated by computers. Synthetic data existed well before the recent AI boom, but GenAI is making it easier than ever to create it.
Major players in the AI space are leveraging synthetic data. Meta has its Self-Taught Evaluator. Google has described its approach to generating private synthetic training data. NVIDIA released a family of open models that users can leverage to create synthetic data for training LLMs.
What are the benefits and risks of synthetic data? What could its future in the AI space look like?
The Benefits of Synthetic Data
Collecting and managing real-world data is expensive. Enterprises need to undertake that costly process themselves or purchase data sets from an outside vendor. With the capabilities of GenAI, companies can now leverage their existing data sets to create rich, synthetic variations.
“It can also help with that manual process of going in and labeling the data,” Kjell Carlsson, head of AI strategy at enterprise AI platform Domino Data Lab, explains. “You often need very talented, expensive … people to go in and do that. You can offload a lot of that work now to these models and do it effectively if not for free, very, very cheaply.”
Synthetic data can also help AI model users avoid a potential minefield: bias.
“All of these of generative AI models or AI bots that have tended to say things that are racist or hallucinate false facts, we can actually use synthetic data to address that problem,” says Erik Brown, senior partner at digital services firm West Monroe. “We can take the bias of actual data out of model training data.”
The process of gathering and using real-world data to train AI models is also rife with privacy concerns. Synthetic data can create representations of individuals’ data without exposing the personal information of real people.
Synthetic data could also provide companies with some legal protection when training their LLMs. The use of copyrighted material for AI model training is highly contentious and a matter that is being fought over in court. In one high profile case, The New York Times is suing OpenAI and Microsoft over the use of its copyrighted material.
The outcome of that lawsuit is not certain, but it is possible that companies will need to license copyrighted material for training LLMs, adding more restrictions and expenses to their data usage. Synthetic data could be an attractive, less expensive option with less legal risk.
“If we start with the notion that synthetic data is created by a machine, then the idea of training a secondary AI system with synthetic data, the implication is that would not violate anyone's particular copyright or other IP rights,” says Geoffrey Lottenberg, partner and lead of intellectual property and technology group at business law firm Berger Singerman.
While massive LLMs and their data needs feature prominently in discussions of the AI space, synthetic data can also be a useful tool for training smaller, more specialized models.
Enterprises can leverage larger LLMs to create synthetic versions of their own data sets, which can then be used to train their smaller, specialized AI models.
“I'm going to use one of those larger models to go in and create synthetic versions of my small data set and then use that synthetic data set, which is now much larger but based on my own data to train this smaller specialized … generative AI model,” says Carlsson.
This approach will “… be cheaper, faster, and more fine-tuned to my area,” he adds.
The Risks
Realizing the benefits of synthetic data is not a given. Users must still concern themselves with the quality of the data they are using.
“Provenance and lineage start to become important because as we move further and further to using AI everywhere, you have to know the quality of the data going in to be able to trust the output,” says Hazard. Enterprises need to know where data comes from and how it has been transformed following its consumption by various systems and models.
If AI model users do not know that information, they cannot ascertain the quality of the data. And that’s where the problems start. Synthetic data can solve issues like bias and privacy, but it can also exacerbate them.
“You don't instantly get rid of the privacy risks when using synthetic data. You do need to know what you're doing, and you do need to test and validate the data sets that you create,” Carlsson warns. “If your data is already biased [and] you're creating a synthetic version of that, effectively you can amplify the biases in your data.”
Synthetic data does not completely erase any possibility of legal risk either. Privacy violations expose companies to legal risk, as does the use of copyrighted material.
“Where does this synthetic data come from? Are we sure it's created purely by machine?” Lottenberg asks.
An IP rightsholder may be able to argue infringement if they determine synthetic data does somehow contain their copyrightable material.
And as with an AI model, trained synthetic data or not, the way the outcomes are used in the real world have potential legal implications.
“If an AI system is created based on synthetic data and as a result of the training it's not all that reliable and some sort of big decision is made, there could be liability for any number of other legal violations,” Lottenberg points out.
One of the buzziest risks associated with the use of synthetic data is model collapse. If AI models are continuously trained on data created by AI, they could potentially become less and less reliable. The cycle of AI models ingesting only content created by AI conjures images of a snake devouring its own tail. In this case, the snake would continue to devour until it only spits out gibberish.
A study published in Nature found that “… indiscriminate use of model-generated content in training causes irreversible defects in the resulting models, in which tails of the original content distribution disappear.”
The model collapse described in this study is a topic of debate. Some say it is a likely outcome. Others say the concern is overblown.
“You're … assuming the worst-case scenario for model collapse to happen. That's not to say that it won't happen,” says Carlsson. “We are human. We take shortcuts. There will be people who train models on data without going in and ensuring the quality of that data, and we'll end up creating bad models as part of that.”
Data governance will be essential to prevent model collapse.
“It … comes down to the ability of humans to use the appropriate systems and checks and balances to maintain quality and govern data,” Brown contends. “If we do that right, I think utilizing synthetic data in the future reduces the risk and improves quality over time, overusing the various imperfect sets of data we have in reality today.”
Synthetic Data and the Future of AI Models
It is likely that the use of synthetic data will increase in the AI space. Gartner anticipates that it will outweigh the use of real data in AI models by 2030.
“The use of it is going to grow over time, and if done correctly, [it will] allow us to create more evolved, more powerful, and more numerous models to inform the software that we're building,” Brown predicts.
That potential future seems bright, but the road there is likely to come with a learning curve.
“Mistakes are going to be made almost undoubtedly in the use of synthetic data initially. You're going to forget a key metric that would judge quality of data,” says Brown. “You're going to implement a biased model of some sort or a model that hallucinates maybe more than a previous model did.”
Mistakes may be inevitable, but there will be new ways to combat them. As the use of synthetic data scales, the development of tools for robust quality checks will need to as well.
“Just the same way that we've kept food quality high, we [need to] do the same thing to keep the model quality high,” Hazard argues.
It is also possible that the use of synthetic data will be impacted by a GenAI market correction. As expectations and reality clash, the adoption of synthetic data could slow for a time, as companies figure out how to put actual valuable use cases into practice.
“It's going to be a bit of a bumpy road for folks who [are] the investors in synthetic data before we get to the broader adoption of it,” Carlsson predicts.
If synthetic data does become the future of AI model training, where does that leave real-world data? How can enterprises talk about the use of one or both types of data?
“It should never be a one and done whereby, ‘Great, I've now created a synthetic version of this. I'm done, I'm never going to collect any real-world data again,’” says Carlsson. “That would be terrible; don't do that. It should be a process of ongoing data collection and ongoing validation of your data sets compared to the real-world data that's out there in the ideal case.”
The choice between synthetic and real-world data, or a mix of both, is going to depend on the use case. Enterprise leaders can make that decision by looking at a few different factors. “Look at … quantity of data, the quality of the data, and the confidentiality of that data and use that as a spectrum to determine how much are you going to lean on synthetic data in various cases,” Brown recommends.
About the Author
You May Also Like